Data Science for Marketing
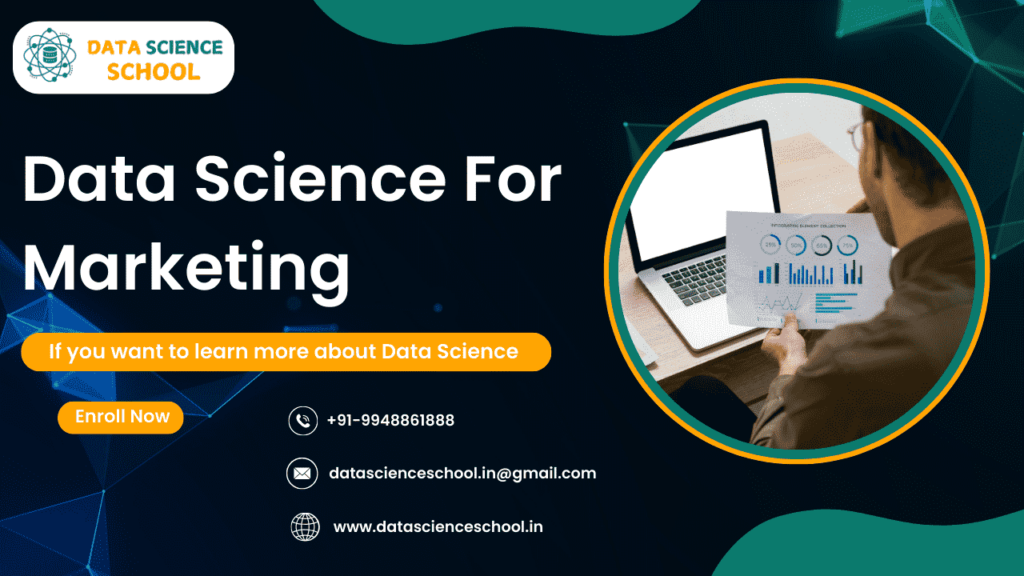
Overview Data Science For Marketing
- Data Science helps marketers understand customer behavior using past data.
- It finds techniques in sales, clicks, and social media activity.
- Companies use data science to predict what customers will buy next.
- It helps in targeting the right audience for ads.
- Marketers can create personalized offers using data insights.
- It improves customer experience by understanding their needs.
- Data science helps in setting the right price for products.
- Businesses use it to measure the success of marketing campaigns.
- It saves time and money by automating marketing decisions.
- With data science, marketing becomes smarter and more effective.
Introduction Data Science for Marketing
Data science for marketing is an emerging field that blends the analytical prowess of data science with the creative aspects of marketing. In simple terms, it involves the use of data driven techniques, including statistical models, machine learning, and predictive analytics, to make smarter marketing decisions and strategies.
In today’s digital world, businesses are generating massive amounts of data through websites, social media platforms, sales data, and customer interactions. The challenge is no longer about collecting this data, but rather how to analyze and make use of it in a meaningful way.
Why is Data Science Important for Marketing?
Marketing is all about understanding customer needs, preferences, and behaviors. The more businesses know about their customers, the better they can meet their needs and create personalized marketing experiences. Data science enables businesses to gain valuable insights from raw data and helps them predict future trends and customer behaviors, allowing them to stay ahead of the competition.
By leveraging data science for marketing, businesses can
- Understand consumer behavior: Through data analysis, marketers can uncover patterns in consumer purchasing behavior, preferences, and spending habits.
- Enhance decision-making: Data-driven insights help marketers make better decisions, leading to more effective marketing strategies.
- Personalize customer experiences: Using customer data, companies can provide tailored marketing messages, personalized offers, and optimized product recommendations.
Why Data Science for Marketing Matters
Marketing in the 21st century is data-driven, and those who fail to adopt data science techniques will likely fall behind. Here’s why data science for marketing matters:
1. Personalization of Customer Experiences
How Personalization Works Using Data Science:
- Recommendation Engines: Platforms like Amazon and Netflix use data science to recommend products and content based on your past behavior. These systems analyze previous purchases, searches, and ratings to suggest new items that are most likely to interest you.
- Targeted Ads: Marketers use data to segment their audience based on demographics, interests, and behaviors, allowing them to serve highly targeted ads. For example, a fashion brand might show a targeted ad for a specific dress to a customer who has previously shown interest in similar products.
- Customer Journey Mapping: By analyzing customer behavior across different touchpoints (website, mobile app, social media), data science enables marketers to create a seamless and personalized customer journey. This means sending the perfect message to the right audience at the ideal moment.
2. Predictive Analytics Shaping Future Marketing Decisions
Predictive analytics is a key component of data science for marketing. It uses historical data to make predictions about future customer behavior, allowing marketers to plan proactive strategies.
How Predictive Analytics Benefits Marketing:
- Forecasting Sales: Predictive models can forecast sales based on trends and seasonal variations. By understanding when demand is likely to increase or decrease, businesses can optimize their inventory and marketing efforts.
- Lead Scoring: In sales, lead scoring refers to assigning a score to potential customers based on how likely they are to convert. By analyzing previous interactions with similar leads, predictive models can score new leads and help sales teams prioritize their efforts.
- Customer Churn Prediction: Predictive models can analyze customer behavior to identify those at risk of leaving. For example, if a customer has not interacted with a brand in several months, they might be at risk of churning. This information enables businesses to take preventive actions, such as sending personalized offers or engaging the customer with targeted content.
3. Customer Segmentation with Data Science
How Data Science Optimizes Segmentation:
- Behavioral Segmentation: By analyzing customer behavior such as website activity, purchase history, and social media engagement, businesses can group customers into segments based on their actions. For instance, a clothing brand might segment customers into categories such as “frequent shoppers,” “bargain hunters,” or “brand loyalists.”
- Demographic Segmentation: Demographic data, such as age, gender, and income, helps marketers understand their target audience and create more targeted campaigns. For example, an online learning platform might target college students with affordable pricing options and working professionals with advanced courses.
- Psychographic Segmentation: Psychographics take into account factors like lifestyle, values, and interests. Data science helps brands understand these deeper aspects of their customers, allowing them to create campaigns that resonate on an emotional level. A wellness brand, for instance, may use psychographic segmentation to target health-conscious individuals who value fitness and wellness.
Challenges in Implementing Data Science for Marketing
1. Data Privacy and Security
With growing concerns over data privacy, businesses must ensure that they comply with data protection regulations such as GDPR, CCPA, and others. Failing to do so can lead to hefty fines and loss of customer trust.
Best Practices for Data Privacy:
- Data Encryption: Protect sensitive customer data by encrypting it both at rest and in transit.
- Anonymization: Anonymizing personal information helps protect privacy while still allowing businesses to use data for analysis.
- Compliance: Marketers need to stay updated on global data privacy laws and implement procedures that ensure compliance.
2. Quality of Data
Data quality is paramount in data science for marketing. If the data collected is incomplete, outdated, or inaccurate, it can lead to flawed insights and misguided marketing strategies.
How to Ensure Data Quality:
- Data Cleaning: Remove duplicate records, fill missing values, and standardize formats to ensure consistency.
- Data Validation: Verify the accuracy and correctness of data before using it for analysis or decision-making.
Future of Data Science in Marketing
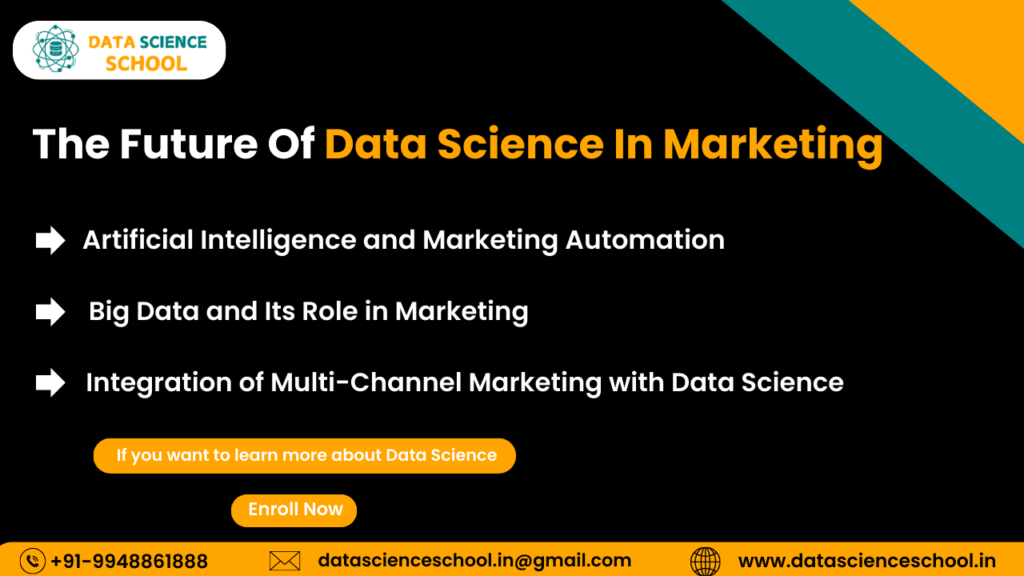
1. Artificial Intelligence and Marketing Automation
Artificial Intelligence is expected to revolutionize marketing by making it smarter, faster, and more personalized. AI-driven tools can analyze vast amounts of data, predict consumer behavior, and automate various marketing processes, reducing the need for human intervention.
AI Applications in Marketing:
- Automated Content Creation: AI-powered tools can generate personalized email marketing campaigns, social media posts, and even product descriptions. By analyzing customer data, AI can create content tailored to each customer’s preferences, increasing engagement and conversion rates.
- Predictive Customer Segmentation: Traditional segmentation relies on demographic and psychographic data, but AI takes it a step further by analyzing behavioral data to predict future trends. For example, AI can segment customers based on the likelihood of them making a purchase, rather than just their current behaviors.
- AI Chatbots: AI chatbots are transforming the way businesses engage with customers. These bots can handle queries, recommend products, and even assist with purchases. As AI algorithms improve, chatbots will become even more sophisticated in understanding and responding to customer needs.
2. Big Data and Its Role in Marketing
Big data refers to the massive volume of data that is generated every second through digital interactions. For marketers, big data provides an unprecedented opportunity to gain insights into customer behavior, preferences, and market trends.
How Big Data Transforms Marketing:
- Real-Time Analytics: Big data allows businesses to analyze customer interactions in real time. This means marketers can react quickly to changes in customer behavior, adjust campaigns on the fly, and optimize customer experiences instantly.
- Customer Sentiment Analysis: With big data tools, marketers can analyze customer sentiment from various sources like social media, online reviews, and customer feedback. By understanding how customers feel about a brand, product, or service, businesses can fine-tune their marketing strategies to address concerns or capitalize on positive sentiment.
- Optimizing Ad Spend: Big data enables businesses to optimize their advertising spend by analyzing performance data across multiple channels. With the help of predictive analytics, businesses can allocate budgets more effectively, targeting the right audience with the right message at the right time.
3. Integration of Multi-Channel Marketing with Data Science
Multi-channel marketing refers to the use of several platforms to engage with customers, such as email, social media, search engines, and more. The challenge lies in integrating data across all these channels to create a unified customer experience.
How Data Science Facilitates Multi-Channel Marketing:
- Unified Customer Profiles: Data science helps marketers integrate data from various sources to build a comprehensive profile of each customer. This unified view allows businesses to provide a consistent experience across channels, whether the customer is browsing the website, engaging on social media, or receiving an email.
- Omni-Channel Strategy: An omni-channel strategy ensures that customers have a seamless experience regardless of which platform they use. Data science enables marketers to analyze customer behavior across touchpoints and optimize campaigns to ensure consistent messaging.
- Real-Time Personalization: By integrating data from multiple channels, businesses can provide real-time personalized recommendations and offers. For example, if a customer abandons a shopping cart on an e-commerce site, they may receive a targeted ad or email with a discount code to encourage them to complete the purchase.
Data Science for Marketing Real-World Examples
1. Personalizing Customer Experiences in E-Commerce
E-commerce businesses have been quick to adopt data science for marketing. Companies like Amazon, eBay, and Alibaba use data science to personalize the shopping experience for each customer.
Amazon’s Recommendation Engine:
Amazon’s recommendation engine is one of the most famous examples of data science in marketing. By analyzing customer behavior such as previous purchases, browsing history, and ratings the system suggests products that are most likely to interest the customer. This not only enhances the user experience but also drives sales by showing relevant products at the right time.
Targeted Marketing by E-commerce Giants:
E-commerce platforms use machine learning to segment their customers based on various factors such as past purchases, browsing behavior, and even time spent on specific pages. By doing so, they can send targeted promotions or product recommendations that resonate with each customer.
2. How Healthcare Uses Data Science to Improve Patient Engagement
In the healthcare industry, data science for marketing is being used to engage patients and drive healthier behaviors. Healthcare providers analyze patient data to send personalized health tips, reminders for check-ups, and even recommend treatments based on past medical history.
Predictive Analytics in Healthcare Marketing:
Using predictive analytics, healthcare providers can identify patients who are at risk of certain diseases or conditions and send proactive health reminders or targeted advertisements for wellness products. By using this data, healthcare marketers can increase patient engagement and improve patient outcomes.
3. How Retail is Optimizing In-Store and Online Experiences
Retailers use data science to bridge the gap between online and in-store shopping experiences. Through data collection in both environments, retailers can create a more seamless experience for customers.
Walmart’s Use of Data Science:
Walmart uses data science to analyze customer shopping patterns and optimize inventory management. By analyzing purchasing data from both online and brick-and-mortar stores, Walmart can forecast demand, stock popular products, and ensure customers have a positive shopping experience, whether in-store or online.
How to Get Started with Data Science for Marketing
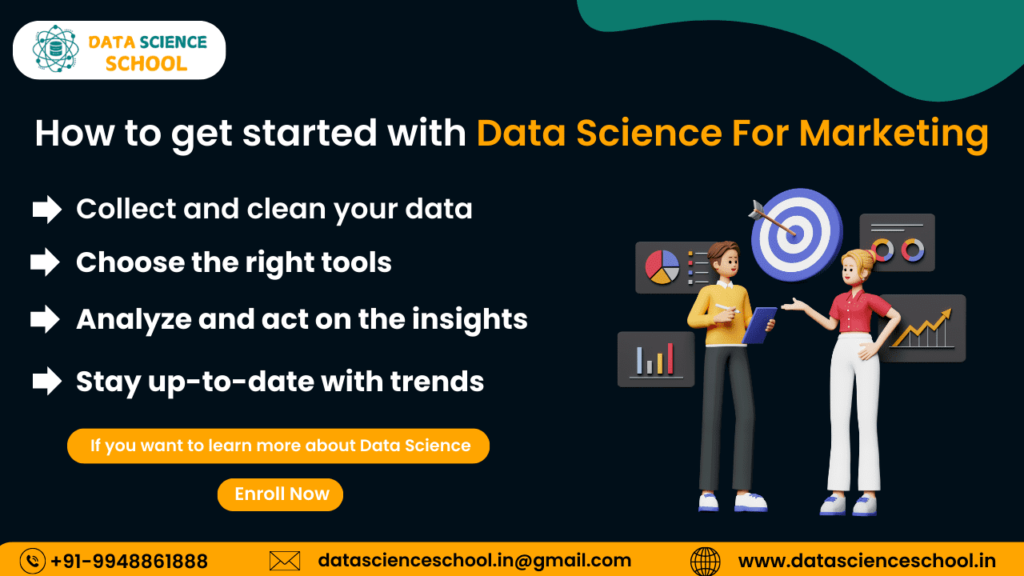
1. Collect and Clean Your Data
The first step in implementing data science for marketing is to collect data from all available touchpoints whether it’s your website, social media, customer service interactions, or sales data. Clean and organize this data to ensure its quality and accuracy.
2. Choose the Right Tools
There are many tools available for marketing analytics and data science, ranging from basic analytics platforms like Google Analytics to more advanced tools like Tableau and R. Choose the tools that best fit your business needs and budget.
3. Analyze and Act on the Insights
Once you’ve gathered and cleaned your data, it’s time to analyze it. Use statistical models, machine learning algorithms, and other analytical techniques to identify trends, predict customer behavior, and uncover insights. Then, apply these insights to optimize your marketing campaigns.
4. Stay Up-to-Date with Trends
Data science and marketing technologies evolve rapidly. Stay up-to-date with the latest trends in Artificial Intelligence, machine learning, and data science tools. Attend webinars, take courses, and follow industry blogs to ensure you are using the best practices.
Challenges in Using Data Science for Marketing
1. Data Privacy Concerns
One of the most significant challenges in using data science for marketing is ensuring that customer data is handled with care and in compliance with privacy laws. Regulations like GDPR (General Data Protection Regulation) and CCPA (California Consumer Privacy Act) have imposed strict guidelines on how customer data should be collected, stored, and used.
How to Overcome This Challenge:
- Ensure Transparency: Marketers need to be transparent with customers about the data they are collecting and how it will be used.
- Anonymize Data: Use anonymization techniques to protect sensitive customer information.
- Comply with Regulations: Stay updated on the latest data privacy laws and ensure that your data collection and usage practices are compliant.
2. Data Quality Issues
Data science relies heavily on the quality of data, and poor-quality data can lead to inaccurate insights and misguided marketing decisions. Data quality issues can arise due to incomplete data, outdated information, or incorrect data entries.
How to Overcome This Challenge:
- Data Cleaning: Implement data cleaning practices to ensure that the data being used is accurate, complete, and up-to-date.
- Invest in Good Data Collection Tools: Use reliable and advanced tools to collect and store data in an organized manner.
- Regular Audits: Perform regular audits of your data to identify and fix any quality issues.
3. Skill Gap
Data science is a highly specialized field, and many marketing professionals may lack the necessary skills to effectively analyze and interpret data. Hiring skilled data scientists or training your marketing team is crucial to leveraging data science in marketing.
How to Overcome This Challenge:
- Hire Skilled Professionals: Consider hiring data scientists or analysts who have experience in applying data science to marketing.
- Training and Upskilling: Provide training to your marketing team so that they can understand and make the best use of data science tools.
- Collaborate with Experts: If you lack internal resources, you can collaborate with external agencies or consultants who specialize in data science for marketing.
4. Integration with Existing Systems
Integrating data science into existing marketing systems and processes can be complex. Many organizations use legacy systems that may not be compatible with modern data science tools and technologies.
How to Overcome This Challenge:
- Upgrade Your Infrastructure: Invest in modern systems that can easily integrate with data science tools and technologies.
- Use APIs and Integrations: Leverage APIs and other integration solutions that allow you to connect data science tools with your existing platforms.
- Ensure Scalability: As your data grows, ensure that your systems and tools can scale to handle the increased load.
Key Metrics to Track with Data Science for Marketing
1. Customer Acquisition Cost (CAC)
CAC calculates the expense of gaining a new customer. By using data science, you can analyze which marketing channels and campaigns are most effective at acquiring customers and reduce the cost of acquisition.
2. Return on Investment (ROI)
ROI helps you evaluate the financial effectiveness of your marketing efforts. By using predictive analytics and machine learning, you can forecast which strategies are likely to yield the highest ROI, allowing you to allocate your marketing budget more efficiently.
3. Customer Lifetime Value (CLV)
CLV is the predicted revenue that a customer will generate for a business over the course of their relationship with the company. By analyzing past purchase behaviors and customer data, data science can help you predict CLV and focus on retaining high-value customers.
4. Conversion Rate
Conversion rate is the percentage of visitors to your website or landing page who take a desired action, such as making a purchase or signing up for a newsletter. Data science can be used to analyze user behavior on your site and optimize for higher conversion rates.
5. Customer Retention Rate
Customer retention is a key indicator of customer loyalty. By using data science, you can analyze customer behavior and identify factors that influence retention. You can then tailor your marketing efforts to improve customer satisfaction and keep them coming back.
Conclusion Data Science in Marketing
In conclusion, data science for marketing is not just a trend but a fundamental shift in how businesses approach marketing. By leveraging data science tools and techniques, marketers can gain valuable insights, optimize campaigns, and provide a more personalized experience for their customers.
As we move into the future, the role of data science in marketing will only continue to grow, powered by advances in AI, machine learning, and big data analytics. Businesses that embrace data science will be better positioned to stay ahead of the competition, make data-driven decisions, and ultimately achieve greater marketing success.
With the right tools, strategies, and mindset, data science can empower businesses to unlock new opportunities and drive growth in the ever-evolving digital landscape. Whether you are just starting with data science or looking to optimize your current strategies, there has never been a better time to harness the power of data for your marketing efforts.
FAQ’S Data Science For Marketing
1. What is data science for marketing?
Data science for marketing means using data to understand customers and improve marketing strategies.
2. How does data science help in marketing?
It helps find patterns, predict customer behavior, and make better marketing decisions.
3. Can data science improve advertising?
Yes, it helps target the right people with the right ads at the right time.
4. What kind of data is used in marketing?
Data from sales, social media, website visits, and customer feedback is used.
5. How does data science help in customer retention?
It analyzes customer behavior to create personalized offers and improve customer service.
6. Is data science only for big companies?
No, small businesses can also use data science to improve their marketing efforts.
7. Can data science help in pricing strategies?
Yes, it helps set the best price based on demand, competition, and customer behavior.
8. Does data science make marketing automatic?
It helps automate tasks like email marketing, ad placement, and customer segmentation.
9. How does data science help in social media marketing?
It tracks trends, customer engagement, and the best times to post content.
10. Do I need coding skills to use data science in marketing?
Not always. Many tools make it easy to use data science without coding.