Data Science In Banking
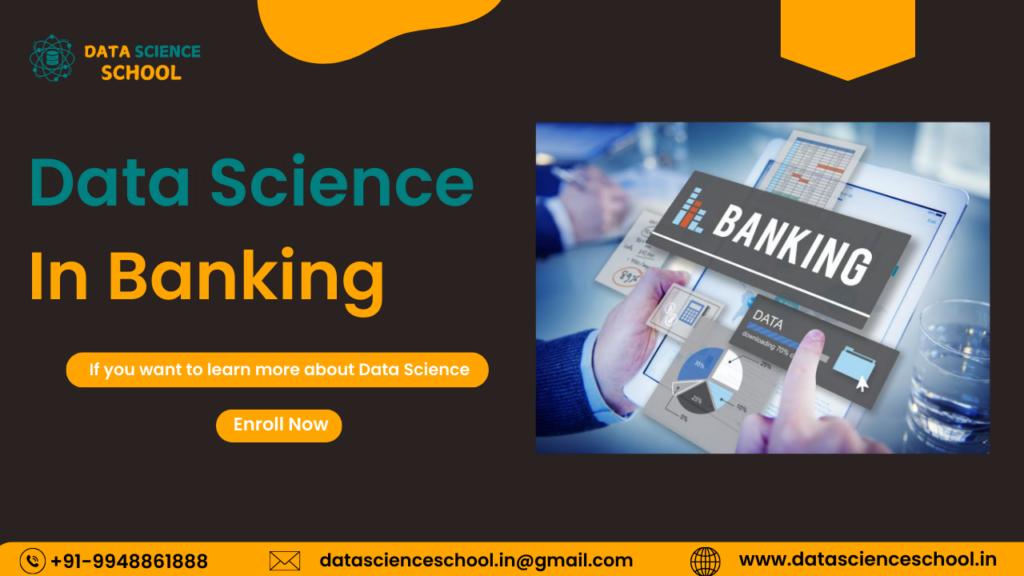
Overview Data Science In Banking
- Stops Fraud: Banks use data to find and stop fraud quickly.
- Personalized Services: Banks suggest financial products based on customer habits.
- Better Loan Decisions: Data helps banks check if a person can repay a loan.
- Faster Loan Approvals: AI makes loan processing quicker and easier.
- Investment Predictions: Banks use data to guess future market trends.
- Keeps Customers Happy: Banks find out which customers might leave and try to keep them.
- Follows Rules & Reduces Risk: Data helps banks follow government rules and avoid problems.
- Fraud Alerts: If a strange payment happens, banks alert customers immediately.
- Chatbots for Help: AI chatbots answer customer questions anytime.
- Better ATM & Bank Management: Banks use data to make sure ATMs have enough cash and branches run smoothly.
Introduction Data Science In Banking
The banking sector in India has changed a lot in the last ten years. With more people using digital payments, mobile banking, and new financial technologies, traditional banking methods are no longer enough. Banks now need smarter ways to serve customers, prevent fraud, and improve operations.
This is where Data Science comes in. It helps banks process huge amounts of data to make better decisions, improve customer service, and keep transactions safe. Data Science is not just about managing data it includes Artificial Intelligence (AI), Machine Learning (ML), Big Data, Blockchain, Cloud Computing, and Natural Language Processing (NLP) to make banking smarter, faster, and more secure.
In this guide, we will discuss how Data Science is transforming banking in India, why it matters, how banks are using it, and the benefits for both banks and customers.
Importance of Data Science in Banking
Data Science In Banking, Data Science is changing banking by helping banks offer better services, detect fraud, manage risks, and work more efficiently. Here are some important ways Data Science is making a big difference in banking:
Enhancing Customer Experience
In today’s fast paced world, customers demand instant and hassle free banking services. Whether it’s checking their account balance, applying for a loan, or making an investment, they want everything at their fingertips.
Personalized Banking: Banks analyze customer data to offer tailor made products, such as customized loans, investment recommendations, and savings plans.
AI-powered chatbots: Many banks, including HDFC, ICICI, and SBI, have integrated AI chatbots to provide instant responses to customer queries, reducing wait times.
Predictive Analysis for Customer Needs: Banks use Machine Learning models to predict customer behavior, ensuring they offer relevant financial products at the right time.
Example: Many Indian banks send personalized credit card and loan offers to customers based on their spending patterns, transaction history, and income levels.
Fraud Detection and Risk Management
Fraudulent transactions and cybercrimes are a major concern for Indian banks. Traditional fraud detection methods depended on manual intervention, which was often slow and inefficient.
- AI-driven fraud detection systems analyze transaction history, spending behavior, and location data to detect suspicious activities in real time.
- Anomaly detection algorithms flag unusual transactions and alert banks instantly.
- Behavioral biometrics such as fingerprint, face recognition, and keystroke dynamics add an extra layer of security to digital banking.
Example: SBI and Axis Bank use AI-powered fraud detection systems to analyze millions of transactions daily and detect fraudulent activities within seconds.
Credit Scoring & Loan Approval
Traditionally, banks assessed loan eligibility based on a fixed set of parameters like salary, credit score, and employment history. However, this method often failed to evaluate people with no prior credit history, such as freelancers or first-time borrowers.
- AI-based credit scoring uses alternative data such as spending habits, utility bill payments, and online shopping behavior to assess creditworthiness.
- Faster loan approvals – AI-powered risk assessment models allow instant loan approvals, reducing the waiting period from weeks to minutes.
- Minimizing bad loans – Predictive analytics helps banks identify potential loan defaulters and take necessary precautions.
Example: Fintech companies like Paytm, Bajaj Finserv, and KreditBee use AI-driven credit scoring to approve loans within minutes, even for people with no credit history.
Algorithmic Trading and Investment Strategies
Data Science is revolutionizing stock trading by enabling banks to make accurate, data-driven investment decisions.
- AI-powered trading bots analyze historical data, market trends, and real-time stock prices to automate stock trading.
- Predictive analytics help investors identify the best stocks to buy/sell, maximizing profits.
- Risk assessment models calculate the probability of market crashes and adjust portfolios accordingly.
Example: ICICI and Kotak Bank use AI-powered investment algorithms to help customers with automated stock trading and wealth management.
Regulatory Compliance and Reporting
Banks must comply with strict RBI regulations regarding financial transactions, risk assessment, and security policies. Checking compliance manually takes a lot of time and can lead to mistakes.
- Automated compliance reporting: AI-powered systems scan financial records and generate compliance reports without human intervention.
- Regulatory risk analysis: Data Science helps banks detect potential violations of RBI guidelines, reducing penalties.
- Early fraud risk detection: Compliance tools monitor suspicious banking activities and prevent fraudulent schemes before they occur.
Example: The RBI’s Data Analytics Unit monitors risk patterns in the Indian banking sector using AI-driven analytics.
Key Benefits of Data Science in Banking
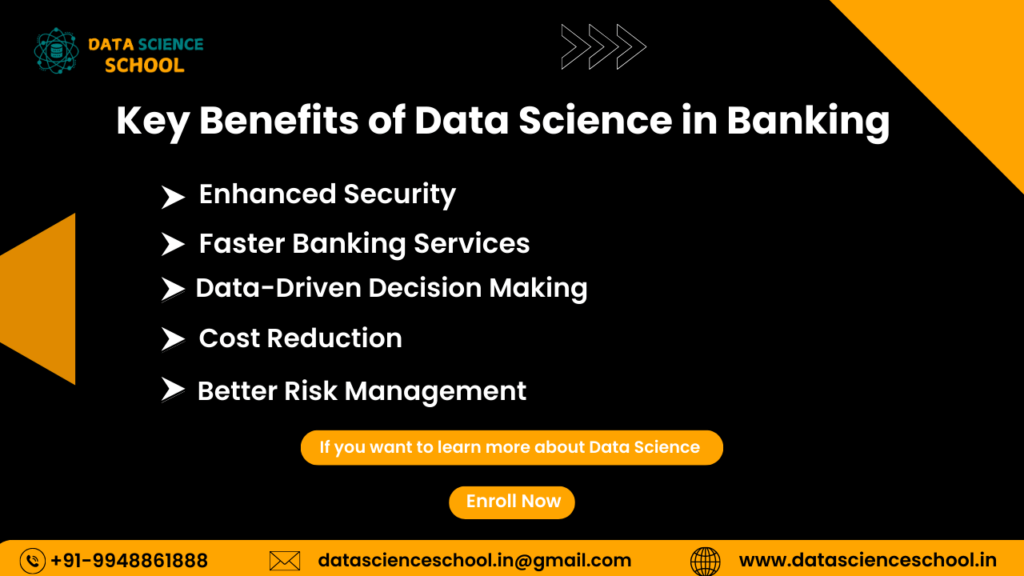
By integrating Data Science and AI, banks experience the following benefits:
- Enhanced Security: AI-driven fraud detection improves cybersecurity and prevents hacking attack
- Faster Banking Services: AI and automation reduce waiting times for customer support, loan approvals, and financial transactions.
- Data-Driven Decision Making: Banks can make smarter investment choices, detect potential risks, and optimize revenue streams.
- Cost Reduction: AI-powered automation reduces the need for manual intervention, cutting down operational costs.
- Better Risk Management: Machine Learning models predict potential market crashes and investment risks, helping banks make proactive decisions.
Technologies & Tools Used in Banking Data Science
As the banking sector embraces digital transformation, several cutting-edge technologies are shaping the future of financial services. From machine learning models detecting fraud to cloud computing enabling faster transactions, Data Science plays a crucial role in optimizing banking operations. Let’s explore some of the most significant tools and technologies powering modern banking.
Machine Learning & Artificial Intelligence The Brain Behind Smart Banking
How AI & ML Are Used in Banking?
Artificial Intelligence (AI) and Machine Learning (ML) have revolutionized banking services by enabling automation, risk assessment, and customer insights. These technologies analyze vast amounts of data to provide personalized financial services and improve operational efficiency.
Key Use Cases of AI & ML in Banking
Use Case | How It Works | Real-World Example |
Fraud Detection | AI-powered systems analyze spending patterns and flag suspicious transactions instantly. | HDFC Bank’s AI model detects unusual activities in real-time, preventing fraud. |
Credit Scoring & Loan Approvals | ML algorithms assess alternative credit data (e.g., mobile bill payments, online shopping behavior). | Bajaj Finserv offers instant loan approvals using AI-based risk assessment. |
Chatbots & Virtual Assistants | AI-powered chatbots provide 24/7 customer support, reducing wait times. | ICICI Bank’s iPal chatbot answers customer queries efficiently. |
Did You Know? AI-powered banks have reported a 30% reduction in operational costs while improving customer satisfaction rates.
Big Data Analytics Power of Customer Insights
What is Big Data Analytics in Banking?
Banks generate petabytes of data daily from transactions, customer interactions, credit reports, and market trends. Big Data Analytics helps banks extract valuable insights to make data-driven decisions.
How Big Data Helps Banks Grow
- Customer Segmentation: Banks group customers based on spending habits and income levels to provide tailored offers.
- Predictive Analytics: Helps in forecasting financial trends and detecting risks.
- Personalized Banking: AI analyzes data to suggest customized investment plans.
Example: SBI uses Big Data Analytics to identify customers who are more likely to default on loans, helping in proactive risk management.
Blockchain Technology Future of Secure Banking
Why is Blockchain Important for Banks?
Blockchain ensures tamper-proof and transparent transactions, making banking more secure and efficient. This technology is widely adopted for cross-border payments, smart contracts, and fraud prevention.
Key Benefits of Blockchain in Banking
- Eliminates Middlemen: Direct transactions reduce processing fees.
- Prevents Fraud: Decentralized records make hacking nearly impossible.
- Enhances Security: Every transaction is encrypted and verified.
Example: ICICI Bank was the first Indian bank to implement blockchain-based international remittances, reducing the processing time from days to hours.
Cloud Computing Is Backbone of Digital Banking
Why Do Banks Use Cloud Computing?
Banks handle massive amounts of sensitive data. Cloud computing platforms like AWS, Microsoft Azure, and Google Cloud help banks securely store, process, and analyze data in real time.
Benefits of Cloud Banking
Feature | Advantage |
Scalability | Banks can expand storage and processing power without investing in physical servers. |
Disaster Recovery | Cloud services ensure zero data loss during system failures. |
Cost-Efficieny | Reduces the need for expensive in-house IT infrastructure. |
Example: Axis Bank uses Microsoft Azure Cloud to power its digital banking services, enabling secure transactions.
Natural Language Processing (NLP) Understanding Customer Sentiments
What is NLP and How Does it Help Banks?
Natural Language Processing (NLP) is a branch of AI that helps banks analyze customer feedback, automate document processing, and improve chatbot interactions.
Use Cases of NLP in Banking
Chatbots & Virtual Assistants: AI-driven chatbots like SBI’s YONO handle routine queries, freeing up human agents.
Sentiment Analysis: NLP analyzes customer complaints to improve banking services.
Document Processing: Banks use NLP to extract information from financial statements and legal documents.
Example: Kotak Mahindra Bank uses NLP-based models to analyze customer sentiments and improve their digital banking experience.
Real-World Case Studies of Data Science in Banking
In India’s rapidly evolving banking sector, Data Science is revolutionizing financial services, improving efficiency, reducing fraud, and enhancing customer experience. Let’s explore real-world case studies of how leading banks and fintech companies in India are successfully implementing Data Science to drive innovation.
Case Study 1: How HDFC Bank Uses AI for Fraud Detection
Challenge
HDFC Bank, one of India’s largest private sector banks, faced a significant challenge in detecting fraudulent transactions in real time. Traditional rule-based fraud detection systems were inefficient, leading to high false positives and delays in response.
Solution
HDFC Bank implemented a machine learning-based fraud detection system that continuously analyzes transactional patterns. The bank used AI-powered anomaly detection models to flag suspicious activities instantly.
Results
- Reduced fraud-related losses by 35% within the first year
- Improved accuracy of fraud detection by 80%, minimizing false alarms
- Real-time alerts for high-risk transactions, ensuring proactive action
Key Takeaway
By leveraging AI and Machine Learning, HDFC Bank significantly enhanced fraud detection capabilities, ensuring secure transactions for millions of customers.
Case Study 2: SBI’s Use of Big Data for Loan Risk Assessment
Challenge
State Bank of India (SBI), the largest public-sector bank in India, faced difficulties in assessing loan applicants’ risk profiles accurately. The traditional credit scoring model relied only on income statements and past credit history, missing out on valuable insights from alternative data sources.
Solution
SBI adopted Big Data Analytics to analyze multiple data points, including:
- Transaction history and spending patterns
- Social media activity and online behavior
- Utility bill payments and digital footprints
Results
- 30% improvement in loan approval accuracy, reducing the risk of defaults
- Faster loan processing, with approvals completed in minutes instead of days
- Increased financial inclusion, allowing more people to access credit
Key Takeaway
SBI’s use of Big Data helped improve credit assessment, allowing better loan decisions and expanding financial accessibility in India.
Case Study 3: ICICI Bank’s Blockchain-Based Cross-Border Transactions
Challenge
Cross-border transactions in India involved lengthy processing times, high costs, and manual verification processes. ICICI Bank wanted to streamline international remittances while ensuring security and compliance with regulations.
Solution
ICICI Bank integrated Blockchain technology into its transaction processing system, ensuring:
- Real-time settlement of cross-border payments
- Transparent and tamper-proof transactions
- Reduced dependency on intermediaries, lowering costs
Results
- Processing time reduced from 2 days to a few hours
- 30% reduction in transaction fees, benefiting businesses and individuals
- Enhanced transparency, minimizing the risk of fraudulent transfers
Key Takeaway
Blockchain technology helped ICICI Bank revolutionize international payments, ensuring faster, cheaper, and more secure transactions.
Case Study 4: Axis Bank’s Cloud-Driven Digital Transformation
Challenge
Axis Bank struggled with scalability issues, slow data processing, and high infrastructure costs while managing its growing digital banking services.
Solution
The bank migrated to Microsoft Azure Cloud, leveraging its computing power, data storage, and AI-driven analytics to enhance digital banking services.
Results
- 80% improvement in transaction processing speed
- Scalability enabled Axis Bank to handle peak traffic efficiently
- Reduced operational costs, saving millions in IT expenses
Key Takeaway
Cloud computing provided Axis Bank with a scalable and cost-effective solution, ensuring seamless digital banking services for customers.
Case Study 5: Kotak Mahindra Bank’s Use of NLP for Customer Sentiment Analysis
Challenge
Kotak Mahindra Bank wanted to improve customer satisfaction by analyzing feedback from emails, social media comments, and call transcripts. Traditional methods were manual and inefficient.
Solution
The bank deployed Natural Language Processing (NLP) models to:
- Analyze customer feedback in real time
- Identify sentiment trends, helping the bank address concerns proactively
- Automate customer support using AI-driven chatbots
Results
- 25% increase in customer satisfaction ratings
- Faster resolution of complaints, reducing response time by 50%
- Improved brand reputation, as negative feedback was addressed more efficiently
Key Takeaway
By using NLP-powered sentiment analysis, Kotak Mahindra Bank transformed customer experience management, ensuring timely resolutions and improved engagement.
The Impact of Data Science on the Indian Banking Sector
Data Science Innovations Are Reshaping Banking
Benefit | Impact |
Fraud Prevention | AI reduces fraud losses and ensures real-time transaction monitoring |
Better Credit Decisions | Big Data allows banks to approve loans faster and more accurately |
Faster Transactions | Blockchain enables near-instant cross-border payments |
Enhanced Customer Experience | NLP helps banks understand and respond to customer needs efficiently |
Cost Savings | Cloud computing minimizes IT infrastructure costs |
Future Trends In Banking Data Science
- AI-Powered Robo-Advisors: Personalized investment recommendations based on real-time data analysis.
- Voice Banking: AI-driven voice assistants enabling hands-free banking transactions.
- Decentralized Finance (DeFi): Blockchain-based banking solutions eliminating intermediaries.
- Hyper-Personalized Banking: Banks using AI and predictive analytics to offer tailored financial products.
The Role of Predictive Analytics In Indian Banking
Predictive analytics is one of the most powerful applications of Data Science in banking, transforming how financial institutions operate. By leveraging historical data, machine learning models, and AI algorithms, banks in India are making data-driven decisions, reducing risks, and offering personalized services.
In this section, we will explore:
- The importance of predictive analytics in Indian banking
- Various use cases where banks benefit from predictive modeling
- Real-world applications showing how Indian banks implement predictive analytics
How Predictive Analytics Is Changing Banking in India
Predictive analytics is helping banks improve efficiency and security across multiple areas. Here’s how:
Function | Impact of Predictive Analytics |
Fraud Detection | Identifies suspicious transactions in real time |
Credit Scoring | Assesses a borrower’s risk profile with high accuracy |
Loan Default Prediction | Forecasts loan repayment behavior to reduce bad debts |
Customer Retention | Predicts which customers might switch banks and helps retain them |
Market Trends Analysis | Forecasts demand for banking products based on customer behavior |
Indian banks are leveraging AI-powered predictive models to increase accuracy, efficiency, and profitability in these domains.
Use Case 1: Predictive Analytics for Fraud Detection in SBI
Challenge
State Bank of India (SBI) struggled with rising cases of online banking fraud, where fraudsters exploited loopholes to conduct unauthorized transactions. Traditional fraud detection mechanisms often failed to catch real-time threats, leading to financial losses.
Solution
SBI integrated AI-driven predictive analytics models into its fraud detection system. These models analyzed:
- Customer spending patterns
- Device and location data
- Unusual login attempts and rapid transactions
Whenever the system detected an anomaly, it immediately:
- Sent real-time alerts to customers
- Blocked suspicious transactions
- Flagged high-risk accounts for further review
Results
- 40% decrease in fraud-related losses
- Faster fraud detection, reducing response time from hours to seconds
- Higher customer trust and improved banking security
Key Takeaway
SBI’s predictive analytics model significantly enhanced fraud prevention, making online banking safer for customers.
Use Case 2: Loan Default Prediction at HDFC Bank
Challenge
HDFC Bank faced issues with rising loan defaults, especially in personal and business loan segments. Traditional credit assessment models were limited and often failed to detect high-risk borrowers.
Solution
HDFC Bank deployed predictive modeling algorithms that analyzed:
- Credit history and transaction behavior
- Employment status and income patterns
- Loan repayment history and spending habits
By using Machine Learning models, the bank identified borrowers with a high probability of defaulting and implemented preventive measures like:
- Offering lower-risk customers better interest rates
- Sending early payment reminders to high-risk borrowers
- Providing financial counseling to customers with repayment difficulties
Results
- 30% reduction in non-performing loans (NPLs)
- Higher approval rates for low-risk borrowers
- Better customer relationships through proactive financial support
Key Takeaway
Predictive analytics helped HDFC Bank make smarter lending decisions, ensuring lower loan defaults and better financial stability.
Use Case 3: Predicting Customer Churn for ICICI Bank
Challenge
ICICI Bank noticed that a significant number of customers were closing their accounts or switching to competitor banks due to service dissatisfaction. The bank needed a data-driven approach to identify at-risk customers and retain them.
Solution
ICICI Bank applied predictive analytics and AI models to:
- Analyze transaction frequency and account activity
- Monitor complaints and negative feedback trends
- Identify customers reducing their interactions with the bank
Based on this data, the bank:
- Offered personalized offers and discounts to retain customers
- Assigned relationship managers to high-risk customers
- Improved customer service touchpoints based on feedback
Results
- 25% decrease in customer churn
- Higher customer satisfaction scores
- Increased retention of high-value clients
Key Takeaway
ICICI Bank used AI-driven analytics to reduce customer attrition, enhancing customer loyalty.
Use Case 4: Market Trends Forecasting by Axis Bank
Challenge
Axis Bank wanted to understand market trends to introduce the right financial products at the right time. They needed a data-driven approach to predict customer demand and market movements.
Solution
Axis Bank implemented Big Data and AI models to:
- Analyze financial market trends using real-time data
- Study economic indicators affecting consumer spending
- Predict demand for banking products like home loans, insurance, and mutual funds
The insights allowed the bank to:
- Launch timely financial products based on demand
- Optimize marketing strategies for specific customer segments
- Provide better investment recommendations to clients
Results
- 20% increase in product adoption rates
- Higher return on investment (ROI) for marketing campaigns
- Improved brand positioning in the market
Key Takeaway
By using predictive analytics, Axis Bank capitalized on market opportunities, ensuring better product positioning.
Future of Predictive Analytics in Indian Banking
The role of predictive analytics in banking is expected to grow significantly in the coming years. Some emerging trends include:
AI-Powered Personal Financial Assistants
Banks will use AI-driven chatbots and virtual assistants that predict spending habits and provide personalized financial advice.
Advanced Credit Scoring with Alternative Data
Instead of relying solely on CIBIL scores, banks will analyze social media behavior, utility bill payments, and e-commerce spending to assess creditworthiness.
AI-Based Risk Management
Banks will use real-time AI models to predict economic downturns, stock market fluctuations, and financial crises, allowing proactive risk management.
Hyper-Personalized Banking Services
Using AI and behavioral analytics, banks will offer tailored financial products, ensuring better customer engagement.
Conclusion Data Science In Banking
- Data Science has transformed banking in India, making it faster, safer, and more customer-friendly.
- Banks use AI and data to detect fraud, predict trends, and make better decisions.
- Advanced technology helps banks offer more secure and personalized services.
- Banks that adopt data science early will have a competitive advantage.
- It helps in better risk management and higher customer retention.
- The future of banking in India will be smart, AI-driven, and highly digital.
FAQ’S Data Science In Banking
1. What role does data science play in the Indian banking sector?
Data science enhances banking operations by enabling data-driven decision-making, improving customer experiences, detecting fraud, and optimizing financial services.
2. How is machine learning applied in Indian banks?
Machine learning is utilized in banking for credit scoring, fraud detection, customer segmentation, and predictive analytics to forecast market trends.
3. What are the benefits of big data analytics for Indian banks?
Big data analytics allows banks to analyze vast amounts of data to gain insights into customer behavior, manage risks, and personalize services.
4. How do Indian banks use predictive analytics?
Banks employ predictive analytics to anticipate customer needs, detect potential fraudulent activities, and assess credit risk.
5. Why is data visualization important in Indian banking?
Data visualization helps banks interpret complex data sets, making it easier to identify trends, monitor performance, and make informed decisions.
6. How is artificial intelligence transforming banking services in India?
Artificial intelligence streamlines banking services through automation, enhances customer interactions with chatbots, and improves decision-making processes.
7. What challenges do Indian banks face in implementing data science?
Challenges include data privacy concerns, integration with legacy systems, the need for skilled personnel, and ensuring data quality.
8. How do Indian banks ensure data security in data science applications?
Banks implement robust encryption, access controls, and compliance with regulatory standards to protect data in data science applications.
9. What is the future of data science in the Indian banking industry?
The future involves increased adoption of AI and machine learning for personalized banking, enhanced fraud detection, and more efficient operations.
10. How does customer segmentation benefit Indian banks through data science?
Data science enables banks to segment customers more effectively, allowing for targeted marketing and improved customer service.