Data Science in Economics
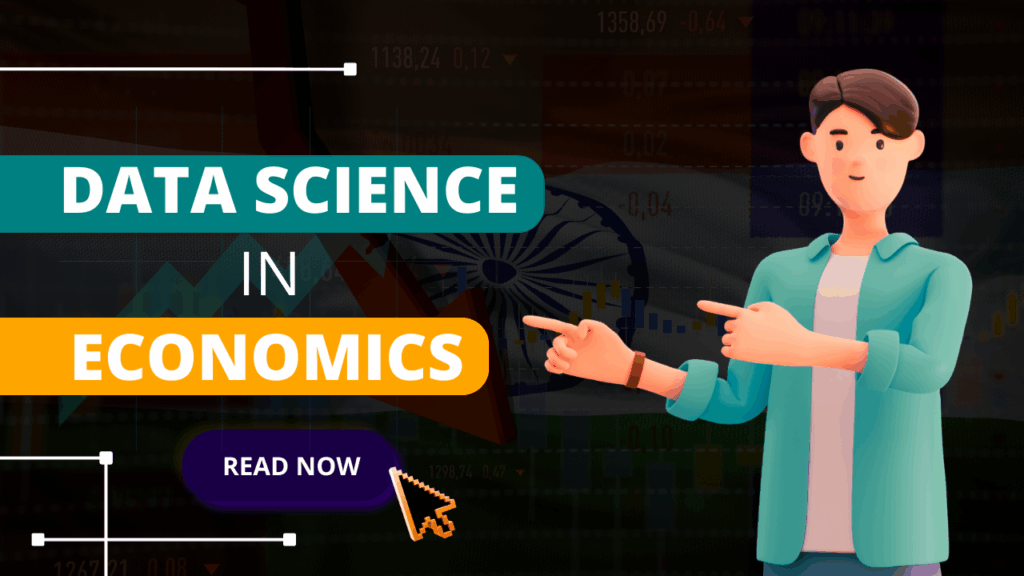
Introduction to Data Science in Economics
Data Science in Economics is one of the most transformative trends in modern economic analysis. With vast amounts of data being generated every second, economists now have a unique opportunity to uncover hidden insights that were once impossible to identify. So, why should you care about Data Science in Economics? Whether you’re an aspiring economist, a business professional, or a data scientist, understanding this intersection can unlock new opportunities for growth and success.
What is Data Science in Economics?
Data Science in Economics merges economic theory with cutting-edge data analysis techniques. Think of it as using technology to make better sense of economic data – from analyzing trends to predicting future market behaviors.
Key Concepts:
Economic data analysis using tools like machine learning and big data.
Predictive analysis of economic outcomes such as inflation, unemployment, and growth.
Optimizing policies with data-driven insights that lead to more efficient decision-making.
Why is Data Science Crucial in Economics?
We live in an era of big data. Without the ability to analyze and process these enormous amounts of information, economists would still be relying on outdated models. Here’s why Data Science in Economics is so important:
Improved Predictions: Predict future trends in real-time.
Better Policy Making: Optimize policies to improve economic outcomes.
Data-Driven Insights: Make informed decisions based on large datasets rather than gut feeling or outdated data.
The Power of Predictive Modeling
What is Predictive Modeling?
In Data Science in Economics, predictive modeling helps economists forecast future events based on historical data. Whether you’re forecasting inflation or predicting consumer spending patterns, this technique is an invaluable tool.
Techniques Involved:
- Regression Analysis
- Time Series Forecasting
- Machine Learning Models
Data Science Tools for Economic Analysis
In the world of Data Science in Economics, certain tools and technologies stand out. Let’s break them down:
Python & R – Most commonly used programming languages for data analysis.
SQL – Helps in managing and querying large economic datasets.
Tableau – A visualization tool that allows economists to present data in interactive formats.These tools help in organizing, analyzing, and visualizing economic data effectively.
Machine Learning: Revolutionizing Economic Forecasting
Machine Learning plays a huge role in Data Science in Economics. It’s not just about understanding data anymore – it’s about predicting outcomes, automating processes, and identifying patterns that were never visible before.
For example:
Classification algorithms help predict which sectors of the economy are likely to grow.
Clustering can identify patterns in consumer behavior, allowing businesses to tailor their offerings more effectively.
Real-World Examples: How Data Science is Changing Economics
Want to know how Data Science in Economics is being applied? Let’s look at some real-world examples:
Stock Market Prediction: Using time series analysis to predict stock prices.
GDP Forecasting: Leveraging big data to project national GDP growth with greater accuracy.
Supply Chain Optimization: Businesses are using data science to minimize costs and optimize inventory management.
Deep Dive into Techniques of Data Science in Economics
Data Science in Economics has proven to be a game-changer, offering more precision, accuracy, and speed in economic analysis. The tools and techniques available today allow economists to look at the world differently, turning massive datasets into actionable insights. Let’s explore these advanced techniques further.
Machine Learning in Economic Forecasting: A New Era
What if you could predict economic conditions with a higher degree of certainty than ever before? Thanks to Data Science in Economics, machine learning models have become one of the most powerful tools for forecasting economic outcomes.
Key Models:
Linear Regression: Used to predict continuous economic variables like GDP growth or unemployment rates.
Decision Trees: These are great for understanding which factors influence economic decisions, like consumer spending or investment behavior.
Neural Networks: Complex algorithms that can predict intricate economic patterns.
Time Series Analysis in Economics
When it comes to Data Science in Economics, time series analysis stands out as a cornerstone for predicting economic trends. By examining historical data points, we can forecast future events in areas like inflation, stock market movements, and trade flows.
Time Series Forecasting:
ARIMA Models (Auto-Regressive Integrated Moving Average): Widely used for short-term economic forecasting.
Seasonal Decomposition of Time Series (STL): Helps in understanding seasonal effects in economic data.
Clustering and Segmentation
Clustering in Data Science in Economics helps to categorize different types of economic entities, such as consumers or regions, based on their behaviors. This technique is valuable for businesses and governments alike.
Common Clustering Techniques:
K-means Clustering: Groups similar economic agents, like customers or regions, based on their purchasing behavior or economic activity.
Hierarchical Clustering: Creates a tree-like structure of data to identify patterns across different economic groups.By segmenting the population, businesses can tailor their marketing strategies, while governments can optimize resource distribution.
Natural Language Processing (NLP) in Economic Analysis
- Did you know that Data Science in Economics isn’t just about numbers? Natural Language Processing (NLP) is revolutionizing how economists analyze unstructured data, such as news articles, social media posts, and economic reports.
Examples of NLP in Economics:
Sentiment Analysis: Understand public opinion on government policies or economic conditions by analyzing news articles and social media.
Topic Modeling: Automatically categorize large volumes of text to detect emerging economic trends.
This technique allows economists to capture insights from sources that were once considered difficult to analyze.
Real-World Applications and Case Studies of Data Science in Economics
We’ve covered the fundamental concepts and techniques, but now it’s time to dive into how Data Science in Economics is actually applied in the real world. In this section, we’ll explore specific case studies and examples to understand how these tools are used to solve economic problems and improve decision-making.
Predicting Stock Market Trends with Data Science
- One of the most exciting applications of Data Science in Economics is stock market prediction. Financial markets are complex systems that rely on vast amounts of data. By applying machine learning and time series analysis, economists and traders can forecast market trends with surprising accuracy.
How It Works:
Sentiment Analysis on financial news articles, tweets, and reports can help gauge market sentiment.
Regression Models predict price movements based on historical data.
Deep Learning Algorithms analyze large datasets to uncover complex patterns.
This combination allows investors to make informed decisions about which stocks to buy, sell, or hold.n
Optimizing Government Policy with Data Science
Governments worldwide use Data Science in Economics to craft policies that are effective and sustainable. By analyzing data on employment, inflation, income inequality, and more, governments can create targeted interventions that lead to positive economic outcomes.
Example: India’s Demonetization Policy
In 2016, India implemented a controversial demonetization policy aimed at curbing black money. Using data science techniques, economists were able to analyze the effects of demonetization on:
- GDP Growth: Tracking short-term and long-term economic growth patterns.
- Inflation: Analyzing changes in prices of essential goods.
- Consumer Spending: Studying shifts in consumer behavior and spending habits.
While the policy’s success is still debated, the role of data science in analyzing its impact was invaluable.
- GDP Growth: Tracking short-term and long-term economic growth patterns.
Predicting Economic Recessions: Case Study of the 2008 Financial Crisis
The 2008 global financial crisis caught many by surprise. However, economic models driven by Data Science could have potentially predicted the downturn earlier.
Key Methods That Could Have Helped:
- Predictive Models: Using indicators like housing prices, credit default swaps, and unemployment rates to predict downturns.
- Clustering Algorithms: Grouping countries or regions with similar economic characteristics to identify vulnerabilities.
- Sentiment Analysis: Analyzing news articles and social media to track the public’s perception of the economy.
By integrating these techniques, economists could have created more accurate forecasts, potentially reducing the severity of the crisis.
- Predictive Models: Using indicators like housing prices, credit default swaps, and unemployment rates to predict downturns.
Consumer Behavior Analysis for Businesses
Data Science in Economics is not limited to government policy or global finance; it’s also incredibly useful for businesses seeking to understand consumer behavior. By analyzing transaction data, customer demographics, and social media interactions, businesses can tailor their products and marketing strategies to meet consumer needs.
Key Tools for Businesses:
- Predictive Analytics to forecast trends in customer preferences.
- Clustering Algorithms to segment consumers based on purchasing behavior.
- A/B Testing to measure the effectiveness of different marketing strategies.
With these tools, companies can create personalized experiences for their customers, boosting sales and customer satisfaction.
- Predictive Analytics to forecast trends in customer preferences.
Big Data for Economic Development in Emerging Economies
Emerging economies often face unique challenges in economic development. Data Science in Economics offers solutions for countries looking to improve their infrastructure, healthcare systems, and education.
How Data Science is Helping:
- Predicting Resource Needs: Big data helps predict infrastructure needs in rapidly growing urban areas.
- Improving Healthcare: Data analytics can identify health trends and allocate resources more effectively.
- Optimizing Education: By analyzing education data, countries can tailor curricula to match the skills needed in the job market.
In countries like India, where large populations and rapid growth present unique challenges, Data Science in Economics has proven to be a valuable tool for achieving sustainable development.
- Predicting Resource Needs: Big data helps predict infrastructure needs in rapidly growing urban areas.
Environmental Economics and Climate Change Predictions
Another key area where Data Science in Economics is making an impact is in environmental economics. With the world facing urgent climate change challenges, economists are turning to data science to model and predict environmental outcomes, including the economic impact of climate change.
Key Applications:
- Predictive Models to forecast the effects of climate change on agriculture, tourism, and health.
- Carbon Pricing Models to help governments set efficient carbon taxes or cap-and-trade systems.
- Optimization Models to design sustainable energy policies that balance economic growth with environmental preservation.
These tools are helping create policies that are both economically and environmentally sustainable.
- Predictive Models to forecast the effects of climate change on agriculture, tourism, and health.
Digital Economy and E-commerce Growth
The rise of the digital economy has brought new challenges and opportunities. From e-commerce to digital payments, Data Science in Economics is instrumental in understanding the dynamics of this fast-growing sector.
Key Insights:
- Consumer Behavior Analytics: Tracking online behavior to predict which products or services will be in demand.
- Pricing Strategies: Using dynamic pricing models to optimize prices based on consumer demand and competitor prices.
- Supply Chain Optimization: Using real-time data to improve logistics and reduce operational costs.
E-commerce giants like Amazon and Flipkart rely heavily on data science to stay ahead of the competition.
- Consumer Behavior Analytics: Tracking online behavior to predict which products or services will be in demand.
The Future of Data Science in Economics
The field of Data Science in Economics is rapidly evolving, and its potential is far from fully realized. In the coming years, advancements in technology, machine learning, and artificial intelligence (AI) will continue to reshape how we study and understand economics. In this section, we’ll take a look at the future of Data Science in Economics, what innovations we can expect, and how these changes will impact both research and policy.
The Rise of AI in Economic Forecasting
AI is expected to play a central role in the future of Data Science in Economics. By automating complex tasks and making more accurate predictions, AI is transforming economic forecasting.
Expected Impact of AI:
- Real-Time Economic Analysis: AI will help economists provide live insights into economic conditions, such as inflation rates, unemployment trends, and global trade fluctuations.
- More Accurate Forecasts: AI models can process vast amounts of data more quickly and efficiently, providing forecasts with much greater accuracy.
- Automated Policy Recommendations: Governments could use AI to automatically suggest policy changes based on real-time data, improving decision-making.
As AI continues to improve, its ability to analyze data and make predictions will only become more powerful, making it a vital tool for economists.
- Real-Time Economic Analysis: AI will help economists provide live insights into economic conditions, such as inflation rates, unemployment trends, and global trade fluctuations.
Integration of Blockchain and Data Science in Economics
The Emergence of Quantum Computing in Economic Models
Blockchain technology, known for its role in cryptocurrencies, is also starting to make waves in Data Science in Economics. By providing a transparent, secure, and decentralized ledger, blockchain can improve the accuracy of economic data, reduce fraud, and enhance data sharing across borders.
How Blockchain is Changing Economics:
- Secure Data Storage: Blockchain ensures that economic data is stored securely, reducing the risk of tampering and fraud.
- Improved Cross-Border Transactions: With decentralized systems, blockchain can reduce transaction costs in international trade and finance.
- Data Transparency: Economic models can use blockchain to track the flow of money, goods, and services, improving the accuracy of economic measurements.
The potential applications of blockchain in economics are vast, and we are only beginning to scratch the surface.
Quantum computing, still in its early stages, has the potential to revolutionize Data Science in Economics. Quantum computers are able to solve complex mathematical problems much faster than classical computers, which could lead to more sophisticated and accurate economic models.
Quantum Computing’s Potential in Economics:
- Faster Economic Simulations: Quantum computers can simulate economic models with much greater efficiency, allowing for real-time analysis of complex data.
- Optimization of Economic Systems: Quantum algorithms could optimize everything from supply chains to investment portfolios, providing businesses and governments with more precise recommendations.
- Advanced Machine Learning: Quantum machine learning could allow for the development of models that learn from data at an exponentially faster rate than traditional systems.
While quantum computing is still a developing field, its impact on economics could be revolutionary.
- Faster Economic Simulations: Quantum computers can simulate economic models with much greater efficiency, allowing for real-time analysis of complex data.
Data Science in Policy Making
One exciting development in Data Science in Economics is the growing shift towards more personalized, data-driven policy making. Governments are increasingly using data science to create policies that are tailored to the specific needs of individuals, regions, or sectors.
Examples of Personalized Policy Making:
- Targeted Welfare Programs: Using data to design more effective social welfare programs based on individual needs and behavior patterns.
- Personalized Taxation Models: Implementing progressive tax models that adjust to a person’s financial situation in real-time, based on data analytics.
- Localized Economic Plans: Tailoring economic recovery or growth strategies to the unique characteristics of specific regions or communities.
This shift toward data-driven, personalized policies can lead to more effective governance and better outcomes for citizens.
- Targeted Welfare Programs: Using data to design more effective social welfare programs based on individual needs and behavior patterns.
The Role of Big Data in Sustainable Development Goals (SDGs)
The United Nations has set 17 Sustainable Development Goals (SDGs) to address global challenges like poverty, inequality, and climate change. Data Science in Economics will play a pivotal role in achieving these goals by helping governments and organizations make data-driven decisions that ensure sustainable and equitable development.
How Big Data Supports SDGs:
- Tracking Progress in Real-Time: Data science can monitor progress towards SDGs, providing governments and NGOs with up-to-date information on areas like poverty reduction, health improvements, and environmental sustainability.
- Resource Allocation: Big data can optimize how resources are distributed for projects aimed at achieving SDGs, ensuring that funds are used efficiently.
- Predicting Future Needs: By analyzing trends, data science can forecast future challenges related to sustainable development and propose proactive solutions.
The integration of big data into SDG initiatives will be crucial in solving global challenges.
- Tracking Progress in Real-Time: Data science can monitor progress towards SDGs, providing governments and NGOs with up-to-date information on areas like poverty reduction, health improvements, and environmental sustainability.
Future Skills
As Data Science in Economics evolves, so too will the skills required to excel in the field. Aspiring economists will need to be proficient in both traditional economic theory and cutting-edge data science techniques.
Key Skills for Future Economists:
- Programming: Languages like Python, R, and SQL will be essential for analyzing economic data.
- Machine Learning: Understanding machine learning algorithms and how to apply them to economic models will be a valuable skill.
- Data Visualization: The ability to present complex data in a clear and understandable way will be crucial.
- Understanding AI and Blockchain: As these technologies play a bigger role in economics, having a grasp on their principles will set you apart.
The future of economics will demand professionals who are comfortable working with both data and economic theory.
- Yes, data science will have a strong future in 2025 and beyond.
- Companies around the world are investing in making decisions based on data.
- The scope of data science and artificial intelligence is expanding in both public and private sectors.
- Salaries are attractive, job roles are diverse, and the demand for skilled professionals is only increasing.
Conclusion
Data Science in Economics is revolutionizing the way economists analyze and interpret economic data. With the integration of cutting-edge tools such as machine learning, AI, blockchain, and big data, economists now have the ability to make more accurate predictions, develop more effective policies, and gain insights into the complex interactions that drive global economies. As we move into the future, the role of data science will only continue to expand, offering new opportunities for businesses, governments, and individuals alike.
For aspiring economists, mastering Data Science in Economics is becoming increasingly important. The future will demand professionals who are comfortable with both traditional economic theory and advanced data analysis techniques. By embracing these innovations and remaining mindful of the ethical implications, we can ensure that Data Science in Economics contributes to building a more efficient, equitable, and sustainable global economy.
FAQ's On Data Science in Economics
What is Data Science in Economics?
- Data Science in Economics combines economic theories with data analysis techniques like machine learning, statistical modeling, and big data analytics to analyze trends and predict future economic outcomes.
How is Data Science used in economic forecasting?
Data Science in Economics is used in forecasting by utilizing tools like time series analysis, regression models, and machine learning to predict key economic indicators such as GDP growth, inflation, and unemployment rates.
What role does machine learning play in economic analysis?
Machine learning is a vital tool in Data Science in Economics, enabling economists to build predictive models that can forecast economic trends, identify patterns, and automate economic decision-making processes.
Why is big data important in economics?
Big data provides insights into economic behavior at an unprecedented scale. By analyzing large datasets, economists can track economic trends, predict future outcomes, and identify opportunities for growth or risk mitigation.
How does Data Science improve decision-making in economics?
Data Science in Economics improves decision-making by providing data-driven insights. Economic decisions, such as policy-making or business strategies, are more accurate and effective when based on detailed, real-time data analysis.