Difference Between BI and Data Science
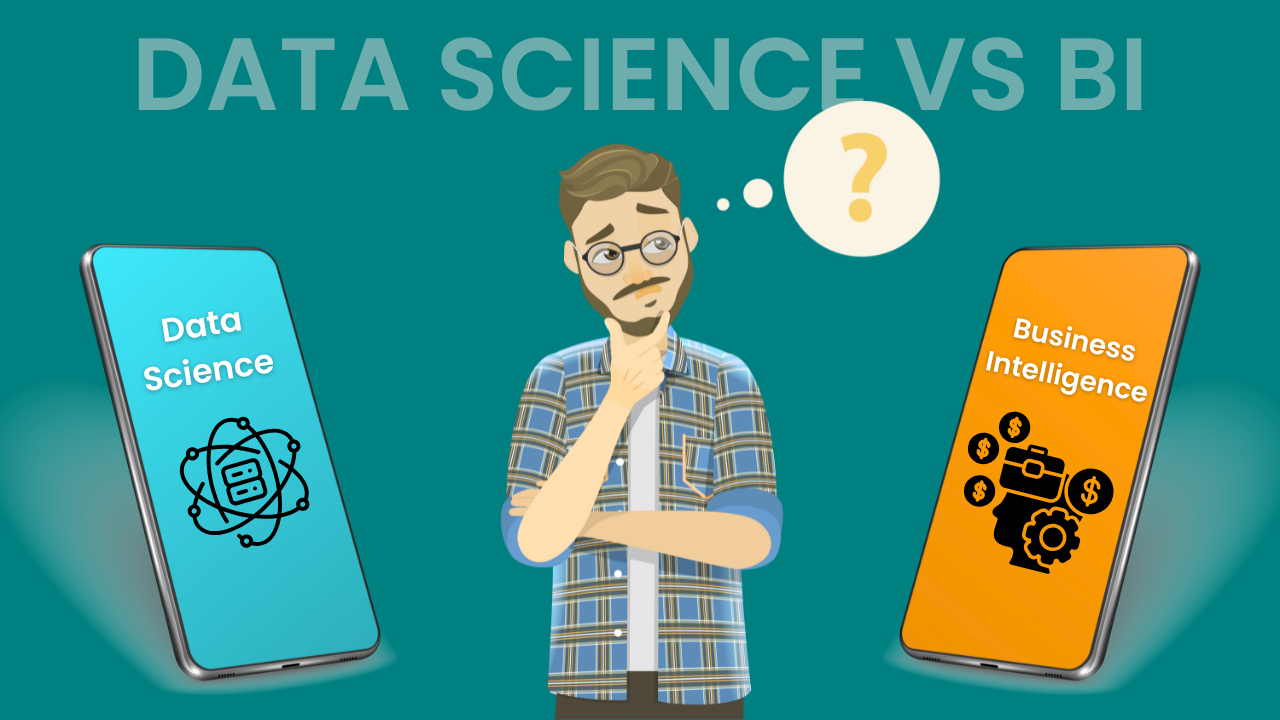
Difference Between BI and Data Science
Introduction
The data-driven world we live in today demands that businesses make informed decisions quickly and accurately. The sheer volume of data available today can overwhelm even the most experienced professionals. However, Business Intelligence (BI) and Data Science have emerged as essential fields for extracting valuable insights from this sea of data. Both disciplines deal with data, but their methodologies and objectives differ, creating a fundamental difference between BI and Data Science.
Business Intelligence (BI) is about using data to understand what has already happened in a business context. It involves collecting data, processing it, and then analyzing it to generate insights that inform strategic decision-making. BI tools focus on turning raw data into actionable information, typically through the use of dashboards, visualizations, and reports. This makes BI a powerful tool for decision-makers, enabling them to assess past performance and optimize business operations.
Data Science, however, takes a different approach. Rather than simply interpreting historical data, Data Science focuses on predicting future trends and behaviors. It uses advanced algorithms, statistical models, and machine learning techniques to not only understand what has happened but also to forecast what will happen. This predictive capability is what sets Data Science apart and makes it an indispensable tool for modern businesses looking to maintain a competitive edge.
The difference between BI and Data Science becomes clearer when you look at their objectives. BI is primarily concerned with descriptive analytics—understanding and interpreting what happened in the past. Data Science, on the other hand, is predictive and prescriptive, helping businesses make decisions based on forecasts and recommendations about the future.
In this article, we will explore these two disciplines in detail, comparing their goals, tools, career paths, salary potential, and more. We will also dive into the practical applications of BI and Data Science, helping you better understand which field may be more suitable for your career aspirations.
Core Definitions BI vs Data Science
To fully appreciate the difference between BI and Data Science, it’s important to understand the core definitions of each field.
Business Intelligence (BI):
Business Intelligence refers to the processes and technologies that organizations use to analyze and present business data. The goal is to help organizations make data-driven decisions by turning raw data into actionable insights. BI tools provide historical, current, and predictive views of business operations, often through dashboards, reports, and data visualizations. The analysis typically focuses on identifying trends, comparing performance, and generating reports that can be used to optimize decision-making.
The role of BI is crucial for understanding the past and identifying areas of improvement. For example, a business might use BI to analyze sales data from the previous quarter to determine which products were most popular. These insights can help shape marketing strategies and improve inventory management.
Data Science:
Data Science, in contrast, is a broader field that involves using scientific methods, algorithms, and systems to extract knowledge and insights from structured and unstructured data. Data Science goes beyond analyzing past data; it focuses on predicting future outcomes and behaviors. It combines elements of statistics, computer science, and domain expertise to build models that can forecast trends, detect anomalies, and provide insights that are not immediately obvious.
Data Science is particularly useful for solving complex problems that require the integration of large datasets from multiple sources. For instance, in e-commerce, Data Science is used to build recommendation engines that suggest products to customers based on their browsing and purchasing history.
Key Differences:
The difference between BI and Data Science lies in the scope and methodology of each field. BI focuses on understanding past and present business performance, while Data Science is more forward-looking, using predictive analytics to forecast future trends. While BI often involves structured data and predefined reports, Data Science often deals with larger, unstructured datasets, applying machine learning models to uncover patterns and trends that can guide future decisions.
Key Differences in Objective
The objectives of BI and Data Science are the key points of differentiation between the two fields. Understanding these distinctions is crucial for anyone looking to pursue a career in either field.
BI: Descriptive Analytics
At its core, Business Intelligence focuses on descriptive analytics. This means that BI is used to describe and summarize the historical and current state of a business. For example, BI can analyze past sales data to identify seasonal trends, track inventory levels, and measure the effectiveness of marketing campaigns.
BI tools often use structured data from sources like databases, spreadsheets, and transactional systems. These tools are designed to turn raw data into clear, digestible information that can inform business decisions. While BI is incredibly powerful for identifying what happened in the past, it does not provide predictions about future outcomes.
The key objective of BI is to provide insights that help businesses improve efficiency, reduce costs, and optimize operations. However, BI doesn’t necessarily answer the question, “What will happen next?” This is where Data Science comes into play.
Data Science: Predictive and Prescriptive Analytics
Data Science, in contrast, is primarily concerned with predictive and prescriptive analytics. Predictive analytics uses statistical models and machine learning algorithms to predict future outcomes. For example, a Data Science team might build a model to predict customer churn based on historical data. This model can then be used to take preventive actions, such as offering targeted promotions to at-risk customers.
Prescriptive analytics, a more advanced aspect of Data Science, suggests possible outcomes and the actions that should be taken. For example, if a company is trying to optimize its supply chain, Data Science can provide prescriptive insights into which suppliers will likely offer the best pricing, delivery times, and reliability.
The goal of Data Science is to provide organizations with actionable predictions and recommendations, helping them anticipate challenges and opportunities before they arise.
Data Science Career Paths vs BI Careers
Choosing between a career in BI or Data Science can be difficult, as both fields offer lucrative opportunities. However, they differ in the types of roles, responsibilities, and skill sets required. Understanding the various Data Science career paths and BI career options can help you make a more informed decision.
Data Science Career Paths
Data Science offers several diverse career paths, each requiring a unique set of skills. Some of the most common Data Science career paths include:
- Data Scientist: Data Scientists analyze complex datasets, build machine learning models, and develop algorithms to make predictions about future trends. They must be skilled in programming languages such as Python or R and be proficient in statistical analysis.
- Data Engineer: Data Engineers are responsible for designing and building the infrastructure that supports data processing and storage. They often work closely with Data Scientists to ensure that the data is in a usable format for analysis.
- Data Analyst: Data Analysts focus on interpreting data, identifying trends, and creating reports. They often bridge the gap between business stakeholders and technical teams by turning data into actionable insights.
- Machine Learning Engineer: A specialized role within Data Science, Machine Learning Engineers design and implement machine learning models and systems that allow companies to automate decision-making and predict outcomes.
BI Career Paths
In contrast, Business Intelligence offers more business-centric roles. Some of the most common BI career paths include:
- BI Analyst: BI Analysts are responsible for analyzing data and creating reports and dashboards that help businesses understand performance and identify opportunities for improvement.
- BI Developer: BI Developers build and maintain the systems that help process, store, and visualize business data. They are skilled in BI tools such as Tableau, Power BI, or QlikView.
- BI Manager: BI Managers oversee BI teams, ensure that data is being used effectively, and coordinate BI projects across departments.
- BI Consultant: BI Consultants help organizations implement BI solutions by providing advice on the best BI tools, strategies, and best practices for data analysis.
Tools and Technologies - BI vs Data Science
The tools and technologies used in Business Intelligence (BI) and Data Science differ significantly due to their different goals and methodologies. While BI tools focus on data visualization and reporting, Data Science tools focus on building predictive models and analyzing large, complex datasets.
BI Tools
Business Intelligence tools are designed for reporting, data visualization, and decision support. Some of the most commonly used BI tools include:
- Tableau: One of the most popular BI tools, Tableau allows users to create interactive and shareable dashboards and reports. It is known for its ease of use and powerful data visualization capabilities.
- Power BI: Developed by Microsoft, Power BI enables users to create rich visualizations and reports from various data sources. It integrates seamlessly with other Microsoft products like Excel and Azure.
- QlikView: A comprehensive BI tool that focuses on data discovery and visualization, QlikView helps users analyze data from different angles to uncover insights.
- SAP BusinessObjects: SAP’s suite of BI tools is designed for enterprise-level analytics, providing reporting, dashboards, and analysis capabilities for large organizations.
Data Science Tools
Data Science tools are designed for deep data analysis, statistical modeling, and machine learning. Some of the most popular tools in Data Science include:
- Python: A versatile programming language used extensively in Data Science, Python has a wide range of libraries for data analysis (e.g., Pandas, NumPy), machine learning (e.g., Scikit-learn, TensorFlow), and data visualization (e.g., Matplotlib, Seaborn).
- R: A programming language specifically designed for statistical computing, R is widely used by Data Scientists for data manipulation, visualization, and modeling.
- Jupyter Notebooks: Jupyter is a popular open-source web application for creating and sharing documents that contain live code, equations, visualizations, and narrative text.
- TensorFlow: An open-source machine learning framework developed by Google, TensorFlow is used for developing and deploying machine learning models, particularly deep learning models.
- Hadoop: A framework for distributed storage and processing of large datasets, Hadoop is used extensively in Data Science to manage and analyze big data.
Data Science Interview Questions vs BI Interviews
One of the most significant differences between BI and Data Science lies in the types of questions asked during interviews for each field. While both fields require a solid understanding of data analysis and business operations, the focus of the interview questions differs.
Data Science Interview Questions
Data Science interviews are heavily focused on technical skills, including programming, statistics, and machine learning. Some common Data Science interview questions might include:
- What is the difference between supervised and unsupervised learning?
- How do you handle missing data in a dataset?
- Can you explain the concept of overfitting in machine learning?
- What is a confusion matrix, and how is it used in classification problems?
BI Interview Questions
In contrast, BI interviews are more focused on the candidate’s ability to use BI tools and interpret business data. Common BI interview questions might include:
- How would you create a dashboard to monitor key performance indicators (KPIs)?
- What is the process for integrating data from multiple sources into a BI tool?
- How do you ensure the accuracy and reliability of business data?
- What is the importance of data visualization in decision-making?
The difference between BI and Data Science interviews lies in the emphasis on programming and machine learning for Data Science roles, while BI interviews focus more on business acumen, data visualization, and reporting skills.
Salary Comparison: Data Science Engineer Salary in India vs BI Professionals
Salaries in Data Science and BI are influenced by several factors, including the specific role, years of experience, and geographic location. In India, the salary difference between Data Science Engineer salary in India and BI professionals is significant, with Data Science professionals typically earning higher wages.
Data Science Engineer Salary in India
The average salary for a Data Science Engineer in India is higher than that of a BI professional. As of 2024, a Data Science Engineer in India can expect to earn anywhere from INR 8,00,000 to INR 15,00,000 per year, depending on their experience and skill set.
BI Professional Salary in India
BI professionals, such as BI Analysts and BI Developers, earn comparatively lower salaries. The average salary for a BI professional in India ranges from INR 5,00,000 to INR 10,00,000 per year. However, with experience and expertise in popular BI tools like Tableau or Power BI, these professionals can command higher salaries.
The higher salary potential in Data Science is due to the increased demand for professionals with advanced technical skills, such as machine learning, deep learning, and predictive analytics.
Which is Better for Your Career: BI or Data Science?
Choosing between a career in BI or Data Science depends on your interests, skills, and career goals. Both fields offer excellent career opportunities, but they cater to different types of professionals.
Consider Your Interests
If you enjoy working with data to uncover trends and insights from the past, then BI might be the right career path for you. BI is great for those who prefer working with structured data, analyzing performance metrics, and producing reports that influence business decisions.
On the other hand, if you’re passionate about working with advanced statistical models, machine learning, and predictive analytics, then Data Science might be a better fit. Data Science is ideal for individuals who enjoy problem-solving, working with big data, and developing algorithms that forecast future outcomes.
Career Growth Potential
While both fields offer growth potential, Data Science has seen a surge in demand over the past few years. This trend is expected to continue as more businesses leverage data-driven insights to remain competitive. On the other hand, BI professionals are always in demand, but the career growth may be more limited compared to Data Science.
Industry Demand
Industries such as healthcare, finance, e-commerce, and manufacturing are heavily investing in Data Science to leverage predictive analytics and AI. BI, however, remains critical for traditional industries that rely heavily on reporting and monitoring historical data.
Practical Applications of BI and Data Science
Both BI and Data Science have wide-ranging applications across industries. The difference between BI and Data Science is evident when you examine how each field is applied to real-world challenges.
Business Intelligence Applications
BI is commonly used in industries such as retail, banking, and manufacturing, where historical performance data is crucial for decision-making. Companies use BI to track sales trends, customer behavior, and operational efficiency. For example, a retail company might use BI tools to analyze sales data to determine the best time to launch a marketing campaign.
Data Science Applications
Data Science is applied in more complex areas, including predictive analytics, machine learning, and AI. For example, in healthcare, Data Science is used to predict patient outcomes, while in finance, it is used for fraud detection and credit risk modeling.
The Future of BI and Data Science
As the business world continues to embrace AI and machine learning, the future of BI and Data Science will see greater integration between the two fields. Data Science will increasingly be incorporated into BI solutions, allowing businesses to leverage predictive insights alongside historical data. This combination will help organizations make better, more informed decisions, with a stronger emphasis on automation and AI.
Both fields will continue to evolve, with Data Science taking the lead in areas like deep learning, natural language processing, and automation. However, BI will remain an essential part of the data landscape, helping businesses interpret and visualize performance metrics.
Why is BI Important for Businesses?
Business Intelligence (BI) plays a crucial role in helping businesses improve their decision-making by providing actionable insights. Through the use of BI tools, companies can gain a deeper understanding of their operations, customer behavior, and market trends, ultimately leading to improved strategic planning and efficiency.
BI helps businesses monitor key performance indicators (KPIs) in real time, allowing them to adjust strategies quickly in response to market changes. For example, a retail company might use BI to track sales performance across different regions, identifying trends and adapting inventory strategies accordingly. By summarizing complex data into easy-to-understand visualizations, BI allows decision-makers to quickly grasp insights and make informed decisions.
Moreover, BI systems are often integrated with various data sources, including financial records, marketing data, and operational performance data, to provide a comprehensive view of a company’s performance. With the ability to track historical trends, BI tools allow businesses to spot areas for improvement, reduce costs, and optimize overall performance.
The Role of Machine Learning in Data Science
Machine learning (ML) is at the heart of Data Science, enabling professionals to create models that learn from data and make predictions or decisions without being explicitly programmed. The ability to make predictions, identify patterns, and provide automated insights sets Data Science apart from Business Intelligence, which mainly focuses on analyzing historical data.
Machine learning can be classified into various types, including supervised learning, unsupervised learning, and reinforcement learning. Each of these categories has its applications in different industries. For example, supervised learning is used in predictive analytics, where past data is used to predict future outcomes. Unsupervised learning, on the other hand, is often used for clustering, anomaly detection, and other forms of pattern recognition in unlabelled data.
In the world of Data Science, ML allows businesses to move beyond simple reporting to predictive and prescriptive analytics, where the insights provided by the models are actionable. For instance, an e-commerce company might use machine learning algorithms to predict customer preferences and suggest personalized products to increase sales. Similarly, fraud detection systems use ML models to detect irregular patterns in transactions and prevent fraud.
By automating data analysis, machine learning reduces the need for manual intervention and enables Data Scientists to process vast amounts of data more efficiently. This predictive capability is one of the primary reasons Data Science has gained so much traction in recent years.
Which is More Complex: BI or Data Science?
When comparing BI and Data Science, one of the most common questions is which field is more complex. While both fields require strong analytical skills, Data Science is generally considered more complex due to the advanced techniques and technologies it employs.
In Business Intelligence, the focus is on understanding and interpreting historical data to help businesses make decisions. The analysis typically involves structured data and relatively simple statistical methods, such as calculating averages, trends, and basic correlations. BI tools are designed to automate these processes, making it easier for non-technical users to generate reports and visualizations.
In contrast, Data Science requires a deeper understanding of algorithms, machine learning, statistics, and programming. Data Scientists use complex mathematical models, often working with large datasets, to uncover insights that go beyond what can be gleaned from simple analysis. The process often involves cleaning and transforming raw, unstructured data before applying machine learning algorithms to make predictions or classifications.
Moreover, Data Scientists must stay up to date with the latest advancements in machine learning and artificial intelligence, fields that are constantly evolving. This technical complexity, combined with the need for domain expertise, makes Data Science a more challenging field compared to Business Intelligence.
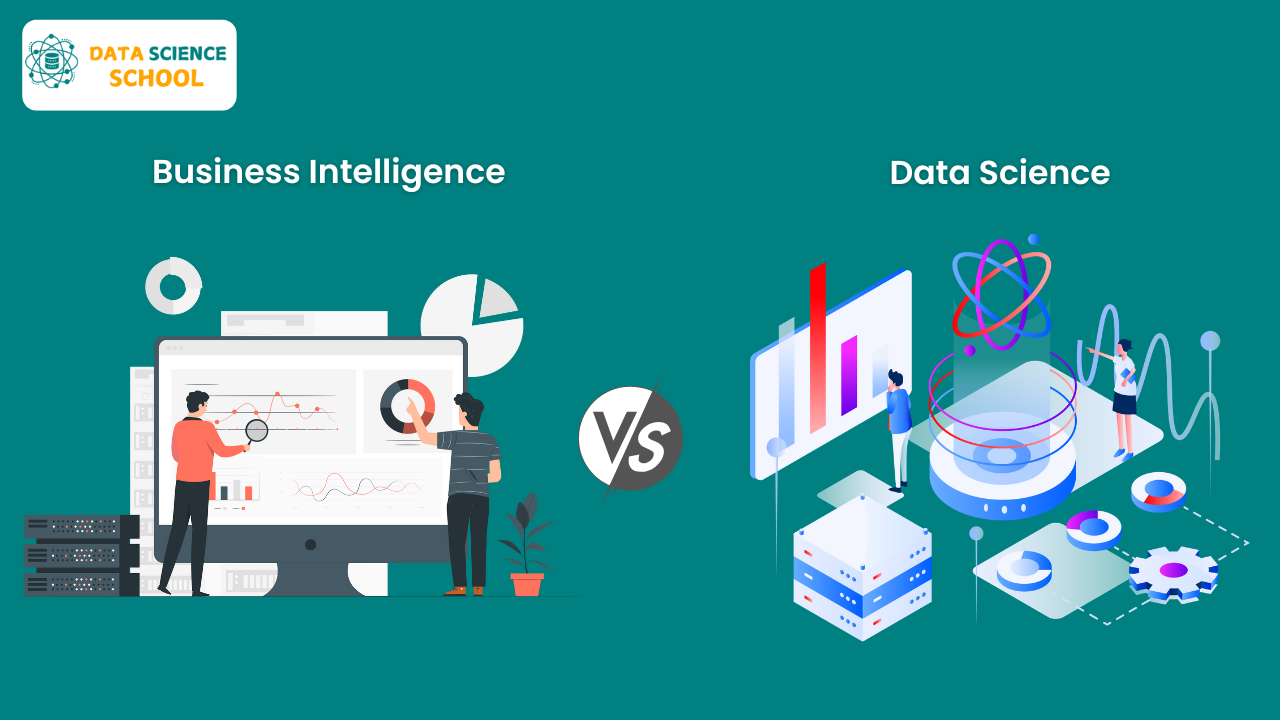
Top 5 Challenges in Business Intelligence and How to Overcome Them
While Business Intelligence offers many benefits, organizations face several challenges when implementing BI solutions. These challenges range from data quality issues to the complexity of integrating multiple data sources. However, these challenges can be addressed with the right strategies and tools.
- Data Quality: Poor-quality data is one of the biggest obstacles to effective BI. Inaccurate, incomplete, or outdated data can lead to incorrect insights and poor decision-making. To overcome this challenge, businesses need to implement robust data governance processes, ensuring data accuracy and consistency.
- Integration of Data Sources: Many organizations struggle to integrate data from various systems, such as ERP, CRM, and third-party applications. BI tools must be able to pull data from multiple sources and present it in a unified view. Using middleware solutions or data warehouses can help overcome this challenge.
- User Adoption: BI tools are only effective if employees use them. Some organizations struggle to encourage adoption across all levels of the business. To improve user adoption, businesses should provide proper training and ensure that the tools are easy to use and aligned with business goals.
- Real-time Data Processing: Traditional BI systems are often slow when it comes to processing real-time data. As businesses require faster insights, investing in modern BI tools with real-time analytics capabilities can address this issue.
- Security and Compliance: As BI tools deal with sensitive business data, ensuring data security and compliance with regulations such as GDPR and HIPAA is critical. Businesses must implement robust security measures and stay up to date with regulatory changes.
How to Transition from BI to Data Science
Many professionals with a background in Business Intelligence are looking to transition into Data Science due to the growing demand for Data Scientists. While both fields deal with data, transitioning from BI to Data Science requires acquiring new skills, knowledge, and experience.
Here are some key steps to make the transition:
- Learn Programming Languages: BI professionals typically have a background in tools like Excel or Tableau, but Data Science requires proficiency in programming languages like Python or R. These languages are used for data manipulation, statistical analysis, and building machine learning models.
- Study Statistics and Machine Learning: Data Science heavily relies on statistical methods and machine learning algorithms. Understanding concepts like hypothesis testing, regression, and classification is crucial for building predictive models.
- Get Hands-on Experience: Practical experience is essential for transitioning to Data Science. Working on real-world projects, such as analyzing large datasets or building machine learning models, will help you gain the necessary experience.
- Take Online Courses and Certifications: Many online platforms offer courses in Data Science and machine learning. Completing certifications from platforms like Coursera, edX, or Udemy can help you acquire the skills needed for a career in Data Science.
- Network with Data Science Professionals: Join Data Science communities, attend webinars, and connect with professionals in the field. Networking can provide insights into the industry and help you stay up to date with the latest trends.
The Impact of AI and Automation on BI and Data Science
Both BI and Data Science are being significantly impacted by Artificial Intelligence (AI) and automation. AI is enabling organizations to gain deeper insights from data and make faster, more accurate decisions.
In BI, AI is used to automate data cleaning, data visualization, and report generation. Machine learning algorithms are being integrated into BI tools to help businesses identify patterns in data and make predictive forecasts. For example, an AI-powered BI tool might automatically generate reports based on the latest sales data and suggest actions for optimization.
In Data Science, AI is being used to develop more sophisticated machine learning models. Deep learning, a subset of machine learning, is enabling Data Scientists to work with unstructured data, such as images and natural language, to solve complex problems in fields like healthcare, finance, and marketing.
As AI continues to evolve, both BI and Data Science will increasingly rely on automation to streamline processes and deliver faster insights.
Top Industries Using BI and Data Science
Business Intelligence (BI) and Data Science are transforming various industries by enabling data-driven decisions and improving operational efficiency. Let’s take a look at some of the top industries that heavily rely on these fields.
1. Healthcare
In healthcare, BI is primarily used to analyze patient data, hospital performance, and operational processes. It helps healthcare organizations optimize resource management, improve patient care, and make data-driven policy decisions. On the other hand, Data Science in healthcare focuses on predictive analytics, such as predicting patient outcomes, optimizing treatment plans, and early detection of diseases through machine learning models. The combination of BI and Data Science helps healthcare organizations reduce costs, improve patient outcomes, and streamline operations.
2. Finance and Banking
The finance sector has long been a leader in BI adoption. BI tools help financial institutions analyze transaction data, track market trends, and manage risks. Data Science, however, is revolutionizing areas like fraud detection, algorithmic trading, and credit scoring. Using predictive models, Data Scientists identify fraudulent patterns and assess creditworthiness more accurately. Financial institutions use both BI and Data Science to gain insights into customer behavior, forecast market movements, and optimize investment strategies.
3. Retail and E-commerce
Retailers and e-commerce businesses are leveraging BI to analyze customer purchasing behavior, inventory management, and sales trends. This helps in streamlining supply chains and optimizing marketing campaigns. Data Science in retail is used for personalized recommendations, demand forecasting, and dynamic pricing models. By integrating machine learning algorithms, retailers can predict customer preferences and offer tailored promotions. Both BI and Data Science are crucial for understanding market trends and delivering a personalized shopping experience.
4. Manufacturing
Manufacturers use BI to monitor production data, track machinery performance, and optimize operational efficiency. Data Science, however, is being employed to improve predictive maintenance, enhance production quality, and forecast demand. By analyzing sensor data from machines, Data Scientists can predict equipment failures and schedule timely maintenance, minimizing downtime. BI helps with reporting and visualization, while Data Science focuses on real-time predictive insights to optimize manufacturing processes.
5. Telecommunications
Telecom companies use BI to monitor network performance, customer satisfaction, and service usage trends. Data Science in telecom focuses on predictive maintenance of network infrastructure, customer churn prediction, and optimizing pricing plans. By leveraging Data Science techniques, telecom companies can better understand customer behavior, personalize offers, and identify potential issues before they impact customers.
6. Marketing and Advertising
In marketing, BI helps track campaign performance, customer demographics, and brand sentiment. It provides insights into past and current marketing strategies to improve future campaigns. Data Science takes it further by using predictive analytics to identify future trends, personalize advertising, and optimize customer targeting. Marketers rely on machine learning algorithms to predict customer lifetime value, optimize content, and enhance conversion rates.
Key Skills for BI Professionals vs Data Scientists
While both Business Intelligence (BI) professionals and Data Scientists work with data, the skills required for these roles differ significantly. Below, we compare the key skills for each profession.
Skills for BI Professionals:
- Data Visualization: One of the core skills of a BI professional is the ability to create meaningful data visualizations. Tools like Tableau, Power BI, and QlikView are used to present data in a user-friendly format, making it easier for stakeholders to make informed decisions.
- SQL Expertise: BI professionals often need to extract data from relational databases, so proficiency in SQL is essential.
- Data Warehousing and ETL: BI professionals need to understand how to organize and process data through ETL (Extract, Transform, Load) processes and data warehousing. This enables them to prepare clean, structured data for analysis.
- Reporting and Analytics: Understanding business KPIs, designing dashboards, and creating detailed reports is crucial for BI professionals. The focus is on descriptive analytics — explaining what happened in the past.
- Excel Expertise: Proficiency in Excel remains an important skill for BI professionals, especially for quick analysis, data cleaning, and reporting.
Skills for Data Scientists:
- Programming: Data Scientists need strong programming skills, particularly in Python and R, which are widely used for data manipulation, analysis, and building predictive models.
- Statistical Analysis: A solid understanding of statistics is essential for Data Scientists to analyze data, develop models, and test hypotheses. They use advanced statistical methods to derive insights from data.
- Machine Learning and AI: Data Scientists must be proficient in machine learning algorithms, such as regression models, decision trees, clustering, and neural networks. Understanding how to train and deploy these models is key to Data Science.
- Big Data Technologies: Familiarity with big data platforms like Hadoop, Spark, and cloud-based data services is important, as Data Scientists often work with large datasets.
- Data Wrangling: Before analyzing data, Data Scientists must clean and preprocess raw data. This includes handling missing values, outliers, and ensuring data consistency.
Key Differences in Skills:
- Focus on Reporting vs Prediction: BI professionals focus more on historical data analysis and creating reports, while Data Scientists concentrate on predictive modeling and data-driven decision-making.
- Toolset: BI professionals typically use BI tools like Power BI and Tableau, while Data Scientists work with programming languages and libraries such as Python, TensorFlow, and Scikit-learn.
Both roles require a strong foundation in data analysis, but Data Scientists often possess more advanced technical skills, especially in programming and machine learning, while BI professionals excel in presenting insights and generating business intelligence through reporting and visualization.
How Data Science is Reshaping Traditional BI Tools
Traditionally, Business Intelligence (BI) tools focused on descriptive analytics, providing historical data and visualizations to help businesses make informed decisions. However, as Data Science evolved, it brought more advanced techniques such as predictive analytics, machine learning, and artificial intelligence (AI) to the forefront, reshaping traditional BI tools.
1. Advanced Predictive Capabilities
Traditional BI tools like Power BI and Tableau primarily focus on data visualization and dashboarding for historical insights. However, Data Science techniques are enabling BI tools to predict future trends using machine learning models. For example, Tableau now integrates with R and Python, allowing data scientists to build machine learning models directly within the platform. These models can predict sales, customer behavior, or inventory needs, helping organizations make proactive decisions.
2. Enhanced Data Integration
Data Science also plays a significant role in enhancing the way BI tools integrate and process data. While BI tools traditionally handled structured data from relational databases, Data Science has expanded this to include unstructured data such as social media content, images, and sensor data. BI tools are now incorporating features that allow for the ingestion of vast amounts of unstructured data and its transformation into actionable insights.
3. Real-Time Analytics
Data Science has driven the shift towards real-time analytics. Traditional BI tools were designed to process batch data and provide periodic reports. With the advent of Data Science, BI tools now incorporate streaming data analytics, providing real-time insights into business operations. This is especially beneficial for industries like finance, retail, and manufacturing, where decisions need to be made in real-time based on live data feeds.
4. Self-Service Analytics
BI tools are becoming more user-friendly, with self-service capabilities that allow business users to generate reports and analyze data without relying on IT teams. However, Data Science has taken this further by integrating advanced analytics and machine learning models into self-service BI tools, enabling non-technical users to apply predictive models and make data-driven forecasts.
5. Automation
Automation is one of the significant trends in both BI and Data Science. BI tools have begun integrating automation features, such as auto-generated reports and AI-driven insights. This allows businesses to scale their data analysis processes without additional human intervention.
Overall, Data Science is pushing BI tools beyond basic data reporting and visualization, empowering businesses with more advanced analytics capabilities, predictive insights, and AI-driven decision-making tools.
The Role of Cloud Computing in BI and Data Science
Cloud computing has become a game-changer in both Business Intelligence (BI) and Data Science by providing scalable, flexible, and cost-effective solutions for storing, processing, and analyzing vast amounts of data. Here’s how cloud computing is transforming these two fields.
1. Scalability and Storage
Cloud platforms like AWS, Microsoft Azure, and Google Cloud offer businesses the ability to scale their data infrastructure as needed. For BI and Data Science, this means that organizations can store large volumes of data and access advanced processing power without the need for on-premise servers. This eliminates the limitations of traditional BI tools, which were often constrained by hardware capacity.
2. Real-Time Data Processing
Cloud computing enables real-time data processing, which is essential for both BI and Data Science. Cloud platforms provide tools for stream processing and analytics, allowing organizations to make decisions based on live data. For example, Amazon Kinesis and Google Dataflow can process massive amounts of data in real-time, empowering businesses to leverage real-time analytics for operational insights.
3. Cost Efficiency
Cloud computing reduces the upfront capital expenses of setting up an on-premise data center. With cloud-based BI and Data Science tools, companies only pay for the resources they use, which can significantly lower costs. Additionally, cloud platforms provide a pay-as-you-go model, offering flexibility for businesses to scale up or down based on their needs.
4. Collaborative Analytics
The cloud enables better collaboration across teams working on BI and Data Science projects. Tools like Google BigQuery, Microsoft Power BI, and AWS QuickSight allow users to work on shared data in a unified environment, improving collaboration and decision-making.
5. Advanced Machine Learning and AI
Cloud computing is also a driving force behind the widespread adoption of machine learning and AI in Data Science. Cloud platforms offer pre-built machine learning models, tools, and frameworks, such as Google TensorFlow, AWS Sage Maker, and Azure Machine Learning. These services allow data scientists to quickly build, train, and deploy machine learning models without needing specialized infrastructure.
6. Security and Compliance
Cloud service providers ensure high levels of security for data stored in the cloud, including encryption, access controls, and compliance with industry standards. This provides peace of mind for businesses adopting cloud-based BI and Data Science tools, ensuring that sensitive data is protected.
Cloud computing has democratized access to powerful BI and Data Science tools, enabling businesses of all sizes to leverage the cloud for faster, scalable, and cost-effective analytics.
Data Science in the Era of Big Data: Challenges and Opportunities
In today’s world, Big Data is shaping how organizations leverage both Business Intelligence (BI) and Data Science. With vast amounts of structured and unstructured data being generated every second, the roles of BI and Data Science have become more critical than ever. However, as data volume, velocity, and variety increase, the challenges associated with handling and analyzing this data also grow.
Challenges in the Era of Big Data:
- Data Quality and Cleaning: The most significant challenge for Data Scientists and BI professionals when dealing with Big Data is ensuring the quality of the data. Raw data from various sources often contains inconsistencies, errors, or missing values that need to be cleaned before analysis. The sheer volume of data makes this process time-consuming and resource-intensive.
- Data Storage and Management: Storing vast amounts of data efficiently and securely is a challenge. With Big Data coming from diverse sources such as IoT devices, social media, or transactional systems, organizations need scalable storage solutions. Cloud computing has played a pivotal role in addressing this challenge, but managing storage costs and ensuring fast retrieval of data remain concerns for both BI and Data Science professionals.
- Data Security and Privacy: As data becomes more valuable, its security is more important than ever. With the growth of Big Data, organizations must adhere to stringent data privacy regulations such as GDPR or HIPAA. Protecting sensitive customer information while ensuring its availability for analysis is a delicate balance that requires careful consideration in both BI and Data Science applications.
- Integration of Data from Multiple Sources: Big Data often comes from disparate sources — cloud databases, on-premise systems, and even social media feeds. Integrating this data to form a cohesive view can be complex. Both BI and Data Science need advanced data integration techniques to merge and analyze data from various sources.
Opportunities in the Era of Big Data:
- Advanced Predictive Analytics: The sheer volume of data offers opportunities for more accurate predictions. By using Data Science techniques such as machine learning, Data Scientists can build models that predict trends, customer behavior, and business outcomes. In BI, this translates into more proactive decision-making, with the ability to anticipate changes and act before they happen.
- Personalization and Targeted Marketing: Both BI and Data Science enable businesses to offer highly personalized experiences to customers. By analyzing customer preferences, past behaviors, and other data points, organizations can create targeted marketing campaigns, product recommendations, and personalized services that drive higher engagement and conversion rates.
- Real-Time Analytics and Insights: With Big Data technologies like stream processing and real-time data pipelines, organizations can access real-time insights that were previously unavailable. This capability allows businesses to respond more quickly to changing market conditions, customer preferences, and operational challenges.
- Automation and AI Integration: Data Science is driving automation through AI and machine learning. With Big Data, it’s possible to train sophisticated AI models that can handle repetitive tasks, analyze vast datasets autonomously, and offer insights that might otherwise be overlooked. This capability is improving business efficiency and decision-making across multiple sectors.
The Ethics of Data Science and BI
As the adoption of Data Science and Business Intelligence (BI) grows, so does the responsibility of handling data ethically. Both fields have a profound impact on businesses and society, which raises ethical concerns related to privacy, data misuse, and algorithmic bias. Here’s a deeper look at the ethical considerations surrounding BI and Data Science.
1. Data Privacy:
One of the primary ethical concerns in both BI and Data Science is data privacy. With vast amounts of personal and sensitive data being collected, organizations must ensure they comply with data protection laws such as GDPR, HIPAA, or CCPA. Data Scientists and BI professionals must adhere to strict privacy guidelines to avoid using data in ways that violate users’ trust. This is particularly important when handling personally identifiable information (PII) or health data.
2. Informed Consent:
Ethical issues arise when data is collected from users without their knowledge or consent. Organizations must inform users about how their data will be used, stored, and analyzed. Transparency is crucial to ensuring ethical practices in both BI and Data Science. Data Science algorithms must be designed to use data in a way that respects individual privacy while delivering insights.
3. Algorithmic Bias:
Both BI and Data Science rely on algorithms to derive insights and make predictions. However, these algorithms are often trained on historical data, which can introduce bias if the data is skewed or incomplete. For example, biased data can lead to biased decisions, such as discrimination in hiring or lending. It’s crucial for Data Scientists to ensure their models are fair and that any inherent biases in the data are identified and corrected.
4. Transparency and Accountability:
As Data Science becomes more integrated into decision-making processes, it’s essential for organizations to maintain transparency in how data is used. Stakeholders, customers, and employees must have a clear understanding of how decisions are made and how data influences those decisions. For example, when Data Science is used in hiring decisions, businesses should be transparent about the criteria used by the algorithms.
5. Ethical Use of Data:
In both BI and Data Science, there is the potential for misuse of data. For example, companies could use data to manipulate consumer behavior, spread misinformation, or create unfair advantages. Ethical BI and Data Science professionals must use data responsibly, ensuring that their work contributes to positive outcomes and that businesses do not exploit consumers or employees.
Conclusion:
The ethics of Data Science and BI are critical for maintaining trust with users and stakeholders. As data-driven technologies evolve, ethical considerations will become even more significant. It’s essential for organizations to implement policies that promote ethical data practices, fairness, and transparency in all BI and Data Science activities.
The Cost of Implementing BI vs Data Science in an Organization
The implementation of Business Intelligence (BI) and Data Science solutions can bring significant benefits to an organization, but both come with their own costs. Understanding these costs is crucial for businesses to make informed decisions about which technology to invest in. Here’s an overview of the costs associated with implementing BI and Data Science solutions.
1. Initial Setup and Infrastructure:
The cost of setting up BI solutions typically includes licensing for BI tools, purchasing hardware (if on-premise solutions are used), and integrating these tools with existing data systems. Popular BI tools like Tableau or Power BI come with licensing fees that can vary based on the number of users or the scale of the organization.
Data Science, on the other hand, requires specialized infrastructure for storing and processing large datasets. This could include cloud services (like AWS, Azure, or Google Cloud), high-performance computing systems, and machine learning platforms. Additionally, organizations need to invest in the software stack (e.g., Python, R, TensorFlow) to support Data Science operations.
2. Software and Tools:
While BI tools often have well-established pricing models (subscription-based or one-time license fees), the cost of Data Science tools is more varied. Many Data Science tools, such as Python and R, are open-source and free. However, advanced machine learning tools, cloud storage, and processing capabilities can become expensive, especially when handling large-scale data projects.
3. Staffing and Expertise:
BI professionals typically require skills in data visualization, reporting, and SQL-based querying. While these skills can be found within many existing IT teams, Data Science requires specialized expertise. Organizations may need to hire data scientists, machine learning engineers, and AI experts who are proficient in statistical analysis, machine learning, and programming. This often leads to higher salaries and recruitment costs for Data Science professionals.
4. Ongoing Maintenance:
Both BI and Data Science solutions require ongoing maintenance, but the scope of this can vary. BI solutions often require less maintenance, primarily revolving around data refreshes and report generation. However, Data Science models require continuous monitoring, updates, and retraining, especially as new data becomes available or business needs change.
5. Return on Investment (ROI):
While the initial costs for Data Science may be higher due to specialized skills and infrastructure, the long-term ROI can be substantial. Data Science models can automate tasks, improve decision-making, and offer insights that can lead to increased revenue or cost savings. BI, while offering substantial value through improved reporting and decision-making, may offer a more incremental ROI.
Conclusion
In conclusion, the difference between BI and Data Science comes down to their objectives and methodologies. BI focuses on understanding the past and making decisions based on historical data, while Data Science is about predicting the future and providing actionable insights for decision-making. Both fields are essential in today’s data-driven world, and your choice between them depends on your interests, skills, and career goals. Whether you choose BI or Data Science, both paths offer tremendous opportunities for growth and success.